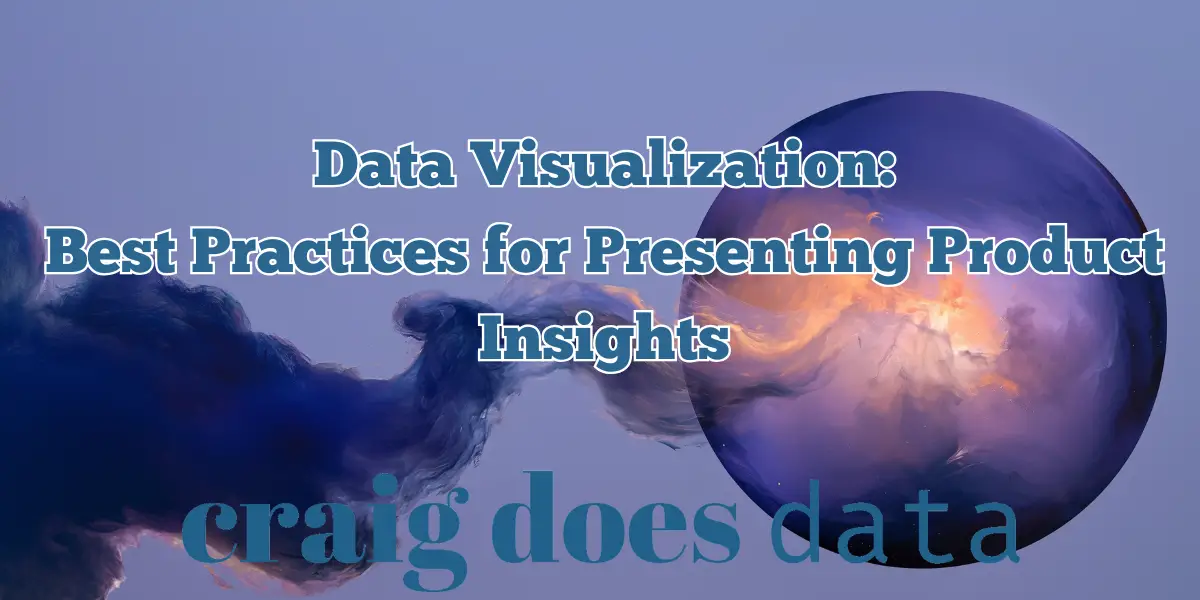
Data Visualization Best Practices for Presenting Product Insights
Introduction
Data visualization has become an essential pillar of modern business strategy, serving as a powerful bridge between complex data sets and the decision-makers who rely on clear insights to guide strategic direction. In an era where organizations accumulate staggering amounts of data, the ability to transform raw numbers and metrics into meaningful visual narratives can drastically influence the success of product development, marketing campaigns, and overall business growth. Effective data visualization not only clarifies intricate patterns but also makes insights more accessible, allowing a broader range of stakeholders—from technical teams to executive leadership—to understand and act on the story that the data tells.
This article aims to explore the best practices for employing data visualization to present product insights. We will delve into fundamental principles, review common pitfalls, and take a forward-looking perspective at the emerging trends and technologies that will shape how organizations visualize and interpret product data in the coming years. Whether you are a seasoned data professional looking to refine your visualization skill set or a newcomer eager to learn the foundations, this comprehensive guide will equip you with actionable strategies to bring clarity and impact to your analytics efforts.
The importance of data visualization for product insights cannot be overstated. From understanding user behavior within digital platforms to uncovering sales trends and market opportunities, visualizations can make or break the realization of a data-driven culture within an organization. By reading this article, you’ll gain an understanding of how to cut through the complexity, design visuals that engage, and create interactive experiences that allow stakeholders to explore data at their own pace. Our goal is to demystify the process, ensuring that everyone involved in product strategy and implementation can leverage the power of visual analytics.
Let’s begin by laying the groundwork with a definition of data visualization and its role in turning product data into reliable, actionable insights.
1. Understanding Data Visualization
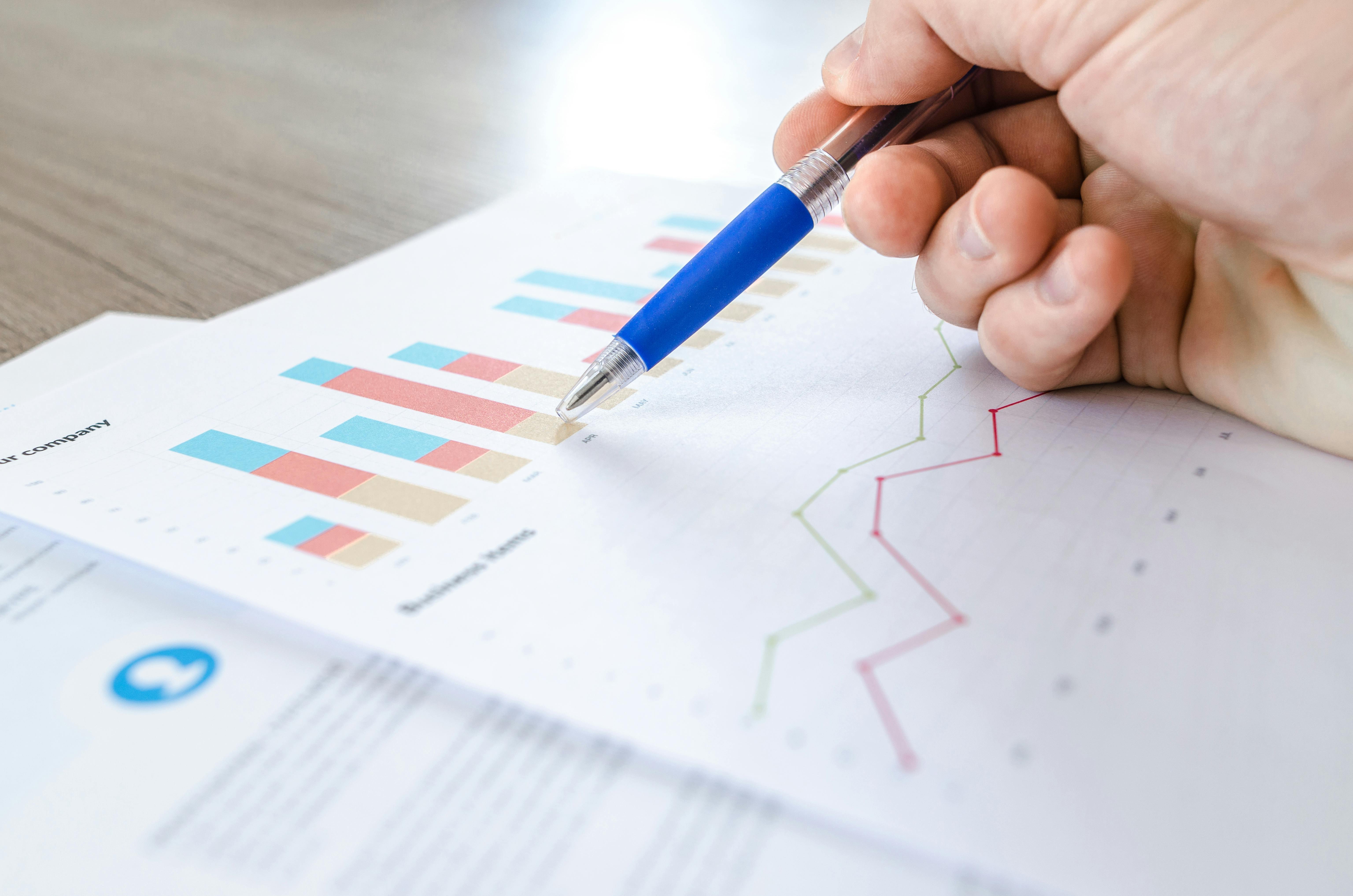
Data visualization is the practice of translating quantitative or qualitative information into graphical formats such as charts, graphs, maps, and infographics. At its core, it serves to illustrate complex data in a way that is visually intuitive, making it easier to detect trends, compare metrics, and draw conclusions. When it comes to product insights, effective visualization enables product managers, UX designers, data analysts, and stakeholders to quickly see how users interact with products, where potential bottlenecks lie, and how different variables correlate with each other.
The role of visualization in data analysis is multifaceted. Firstly, it accelerates understanding by tapping into our innate ability to process visual information rapidly. While reading through spreadsheets full of numbers can be time-consuming and prone to misinterpretation, a well-crafted chart or dashboard can reveal patterns and anomalies at a glance. Secondly, visualization aids in communication. Data analysis is rarely an isolated exercise; instead, it often involves collaboration among multiple teams. Visuals act as a common language through which technical and non-technical stakeholders can converse effectively, reducing ambiguities and misinterpretations.
The importance of data visualization becomes even more pronounced when dealing with product insights. Modern products, whether they are software applications or physical goods, generate diverse data streams such as user engagement metrics, error logs, customer feedback forms, and market performance indicators. Without an organized approach to visualize these data points, it is challenging to prioritize feature rollouts, track user satisfaction, or justify resource allocation. Visualization offers a lens through which critical signals can be identified early, enabling quick course corrections or opportunity seizing.
For instance, consider a SaaS (Software as a Service) product that accumulates user login frequency, session duration, feature usage metrics, and churn rates. If these metrics remain in their raw form (rows in a database or lines in a log file), it’s difficult for even the most experienced data teams to get a holistic view. However, when visualized effectively—for example, using a dashboard that shows line charts for churn over time, bar graphs comparing feature adoption, and funnel charts for user conversion—patterns suddenly emerge. One might notice a spike in churn soon after certain feature releases or see that a newly introduced feature is drastically underutilized. These insights pave the way for data-driven decisions, such as refining a feature or providing better onboarding for new users.
Furthermore, data visualization can serve as a rallying point within an organization. When product insights are distilled into a compelling graphic or interactive dashboard, it encourages broader collaboration. Executives can strategize product roadmaps, marketing teams can fine-tune campaigns, and support teams can anticipate user challenges. This level of accessibility not only fosters a culture of transparency but also drives accountability, as each stakeholder can see how their domain impacts and is impacted by the product’s performance.
In summary, data visualization sits at the intersection of art and science—requiring creativity in design while demanding methodological rigor in data preparation. It democratizes access to insights, speeds up decision-making, and offers a unifying language for diverse teams. As we move forward in this article, we will dissect the critical principles that underpin effective visualization, guide you through the selection of tools, and highlight pitfalls you’ll want to avoid. Remember, the end goal is not merely to create aesthetically pleasing charts, but to produce visual narratives that drive meaningful action in product development and strategy.
2. Key Principles of Effective Data Visualization
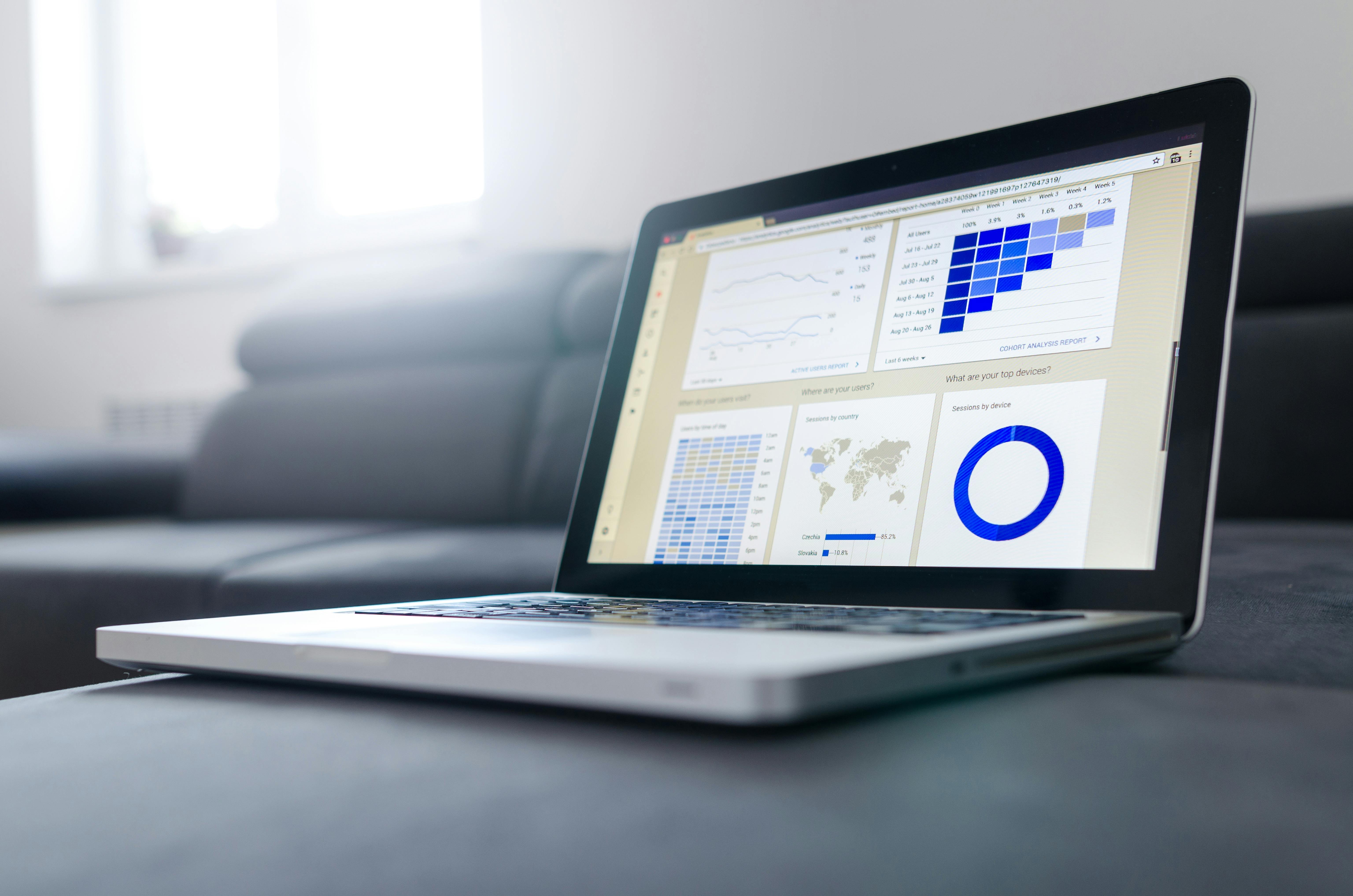
Clarity
Clarity is the bedrock of any impactful visualization. When the message is clear, the audience can directly interpret and internalize the insights. Clarity entails a judicious choice of chart types, axis labels, annotations, and legends. Crowded visuals with overlapping data points, ambiguous color coding, or cryptic labeling risk creating more questions than answers. To maintain clarity, it’s vital to consider the core question you want the visualization to answer. For example, if you aim to show the relationship between two variables, a scatter plot might be the best choice; if you want to compare discrete categories, a bar chart might do the trick.
Another aspect of clarity involves the textual elements surrounding your charts. Label your axes and data points in a way that highlights key figures without cluttering the graphic. Strategic use of tooltips in interactive dashboards can also provide additional detail without overcrowding the primary chart space. When presenting product insights, clarity can ensure stakeholders immediately recognize whether a product’s metrics are improving or if there’s an underlying issue that demands urgent attention.
Simplicity
Simplicity goes hand in hand with clarity. One common misconception is that a complex problem requires an equally complex visualization. In reality, simplicity can be more powerful. A minimalist design that foregrounds the essential data points and eliminates distractions tends to be more effective in guiding the audience to the most critical insights. Ask yourself whether each element of the visualization is contributing to the overall message. If not, consider removing it or simplifying it.
Simplicity also extends to how many data dimensions you attempt to display at once. While 3D charts and multiple combined chart types might look impressive, they can also obscure the main takeaways. Particularly for product insights, you might want to highlight a single key metric—like retention rate—over a specified time horizon. Additional metrics can be shown separately or through interactive features, preserving the simplicity of the primary view.
Accuracy
Accuracy in data visualization is paramount for preserving trust. Even minor misrepresentations, such as truncated axes that exaggerate trends, can lead stakeholders to draw the wrong conclusions. Ensuring your data is well-prepared and your scales and proportions are correct is crucial for credibility. While it may be tempting to manipulate scales to emphasize a positive trend, such shortcuts can backfire by damaging your integrity and leading to misinformed decisions.
Suppose you are visualizing a product’s monthly active user (MAU) growth. If the data is not plotted accurately—for instance, if the baseline of the y-axis starts near the maximum rather than zero—it might falsely indicate dramatic fluctuations. This not only misleads viewers but can cause overreactions in product strategy. Whether your audience is internal or external, always treat data with respect, presenting it in a manner that accurately conveys the underlying reality.
Consistency
Consistency involves maintaining uniform design language across multiple visualizations. This can include using the same color palette, font style, and chart types for similar data categories. Consistent design streamlines comprehension because the audience knows what to expect from your visuals. If you switch between drastically different chart styles and color schemes within a single dashboard or presentation, the viewer may struggle to follow the storyline.
For product insights, consistency might manifest in how you report metrics month-to-month or quarter-to-quarter. Using the same style of bar charts or line charts to display changes in user engagement or revenue can help stakeholders quickly parse and compare different time periods. This uniformity not only establishes visual harmony but also improves the speed and efficiency with which teams can make data-driven decisions. It can be worth implementing standards on this across an organization to promote comprehensibility.
3. Choosing the Right Visualization Tools
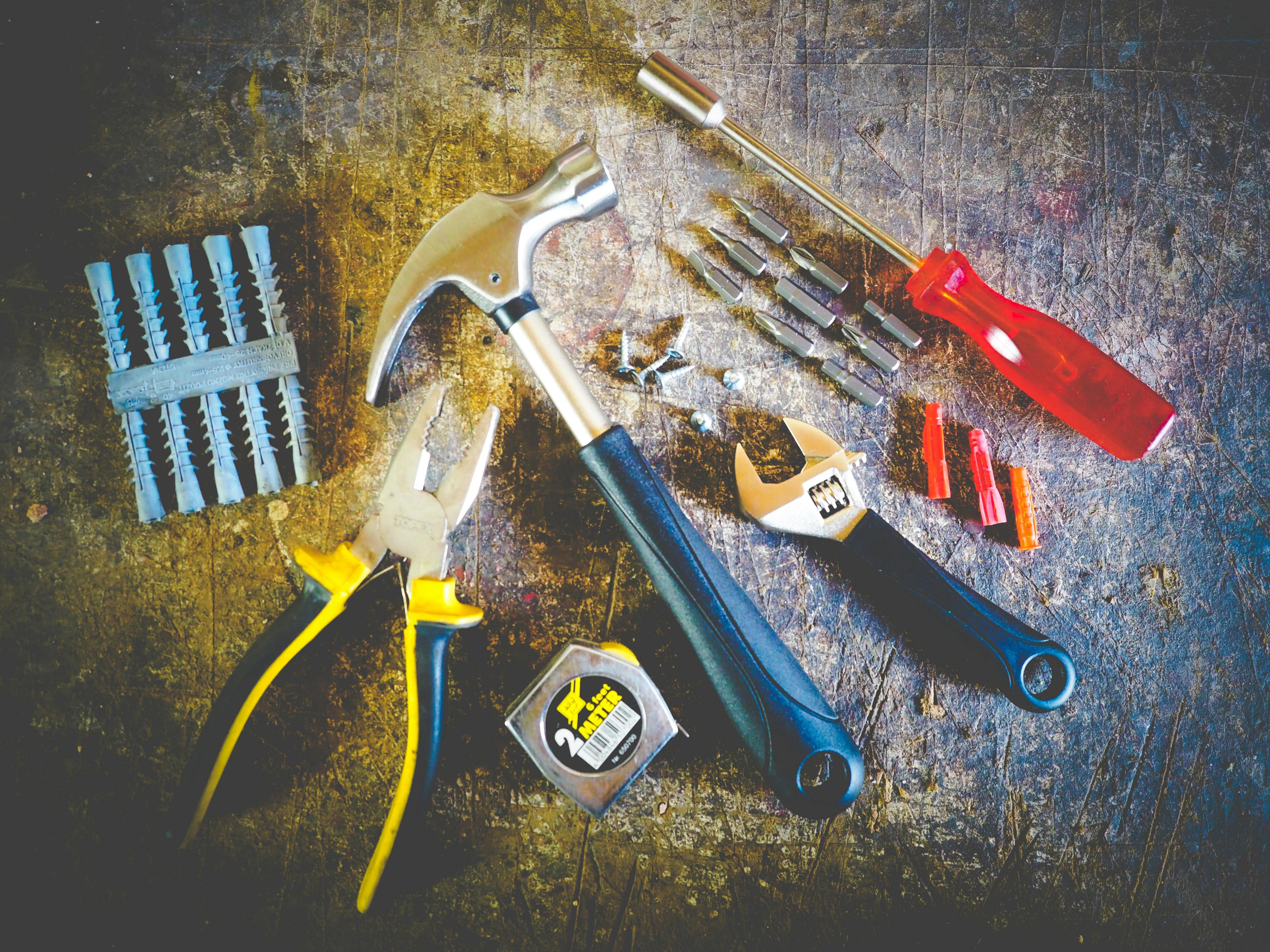
A myriad of data visualization tools are available in the market, each catering to different levels of expertise, data complexities, and organizational needs. Selecting the right tool is a critical step that can determine the success of your product insights initiatives. Some tools offer user-friendly interfaces that allow non-technical stakeholders to generate charts with a few clicks, while others provide sophisticated libraries and programming environments for advanced customization.
Tableau is widely regarded for its drag-and-drop interface, which allows you to connect to various data sources and quickly produce insightful dashboards. Its strength lies in its ease of use, robust community support, and an extensive library of visualizations. Power BI, developed by Microsoft, offers similarly intuitive functionalities, with seamless integration into other Microsoft products like Excel and Azure. Businesses heavily invested in the Microsoft ecosystem often find Power BI to be a natural fit.
D3.js (Data-Driven Documents) is an open-source JavaScript library that excels in custom, highly interactive, and visually stunning data representations. It provides granular control over every element of your visualization but comes with a steeper learning curve. For organizations that require highly specialized visual forms or those that want the flexibility of complete customization, D3.js is often the go-to choice.
When evaluating which tool to adopt, consider the size and complexity of your data. If you are handling massive datasets requiring complex transformations and near-real-time analysis, enterprise-grade solutions with strong backend integrations might be necessary. The skill level of your team is another factor; a platform requiring advanced coding skills could be a barrier for non-technical users. Lastly, keep in mind the preferences of your target audience. If you are creating dashboards for executives who only need to view key metrics at a glance, you might opt for a straightforward dashboarding tool. If your audience is more technical and requires deep interactivity or the ability to drill down into the minutiae, you may prioritize advanced interactivity features.
Here is a brief overview of the pros and cons of some popular visualization tools:
- Tableau: Pros: user-friendly, lots of pre-built features, strong community. Cons: license costs can be high, limited advanced customization compared to coding libraries.
- Power BI: Pros: seamless Microsoft integration, affordable for small organizations, user-friendly. Cons: limited custom visuals, sometimes lags in real-time data processing.
- D3.js: Pros: unmatched customization, large developer community. Cons: steep learning curve, longer development time.
- Looker: Pros: built-in data modeling layer, strong collaboration features. Cons: can be expensive, may require more setup time than simpler tools.
Ultimately, no single tool is perfect for all situations. The optimal choice depends on your organization’s data infrastructure, the technical proficiency of your team, the complexity of the product insights you wish to visualize, and the preferences of your stakeholders. Regardless of which tool you choose, the guiding principles of effective data visualization—clarity, simplicity, accuracy, and consistency—remain the same.
4. Best Practices for Designing Visualizations
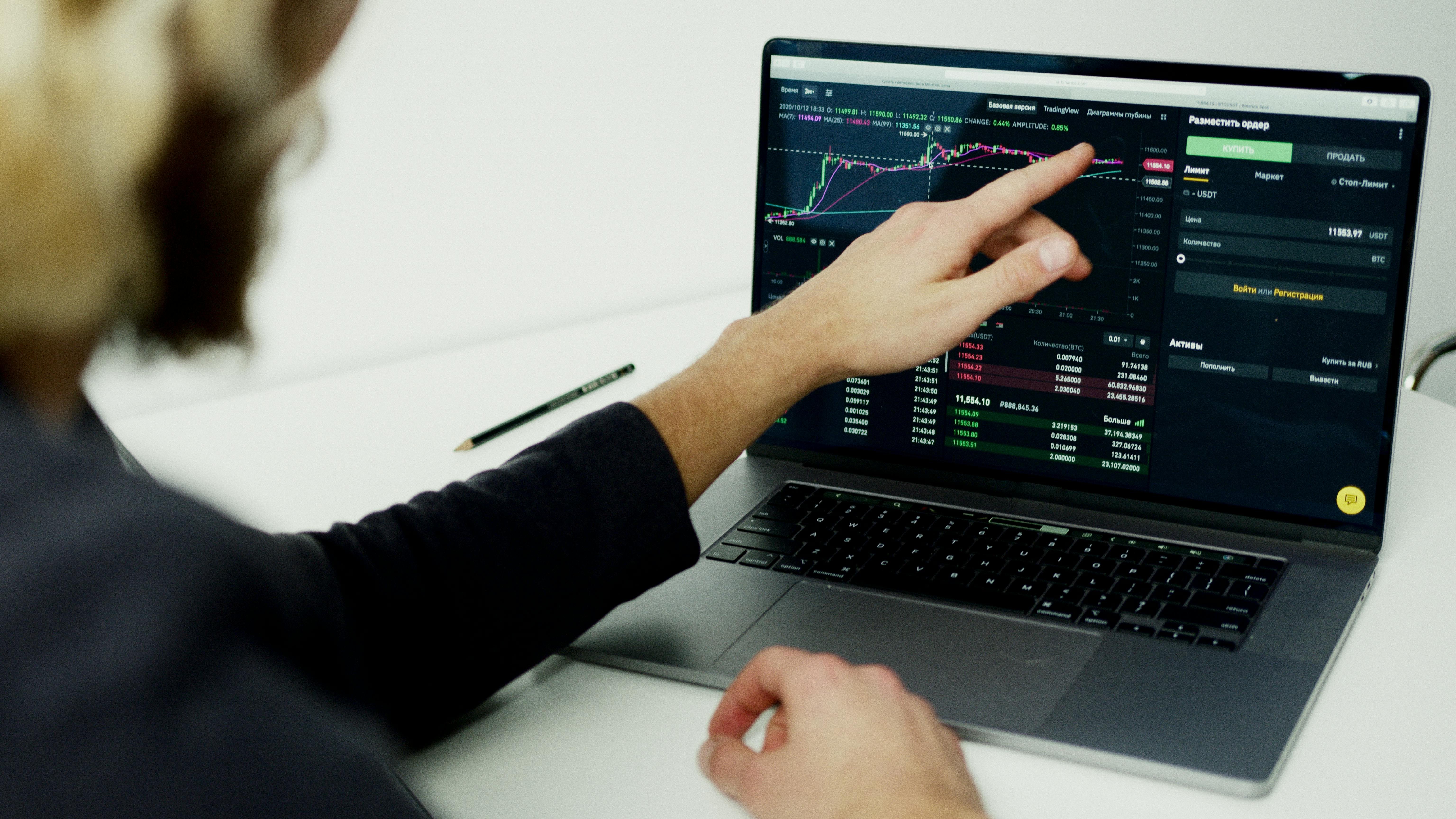
Know Your Audience
Before plotting a single data point, consider who will be viewing your visualization. Are they data-savvy analysts or general managers who need a high-level overview? Understanding your audience’s background and expectations informs decisions about chart types, labeling, and the level of detail to include. For stakeholders unfamiliar with statistical jargon, it may help to use more descriptive labels and offer optional drill-downs rather than bombarding them with complex figures. For teams of data scientists, you can afford to present granular data and more intricate charts because they have the skill set to interpret them correctly.
Additionally, the audience’s objectives should influence the design. An executive presentation focusing on revenue milestones might call for visualizations of monthly recurring revenue (MRR) trends and forecasts, while a product development meeting may require feature usage comparisons and bug report frequencies. By tailoring your visuals to your audience, you increase the likelihood that your insights will drive meaningful action.
Select the Right Chart Type
One of the most common challenges in data visualization is choosing the chart type that best conveys the story. Different chart types excel at highlighting different relationships:
- Bar Charts: Excellent for comparisons among categories (e.g., comparing total sales by product line).
- Line Charts: Ideal for showing trends over time (e.g., daily active users over a month).
- Pie Charts: Useful for representing parts of a whole, although they can become confusing with many slices.
- Scatter Plots: Best for exploring relationships or correlations between two variables (e.g., marketing spend vs. user acquisition rate).
- Funnel Charts: Helpful in illustrating sequential processes and drop-off points (e.g., user signup flow).
Make sure to choose a chart type that makes your message immediately understandable. It’s often more intuitive to compare bars in a bar chart than slices in a pie chart, particularly if you have more than a handful of categories. Similarly, if your aim is to highlight how a metric changes over time, a line chart’s continuous flow is more suitable than scattered columns. Aligning your chart type with your analytical goals significantly enhances comprehension.
Use Color Wisely
Color is a potent tool for directing attention, distinguishing categories, and setting a visual tone. However, it can also be a source of confusion if misapplied. A well-thought-out color palette can quickly guide the viewer to focus on what matters. For instance, if you’re displaying a chart that tracks customer satisfaction levels over time, you might use a single hue that shifts in intensity as satisfaction changes. On the other hand, if you need to compare two distinct metrics—like sign-up rate versus churn rate—contrasting colors can underscore their difference.
Also, be mindful of color-blindness and other visual impairments. Incorporating patterns or textures in addition to colors can be beneficial for ensuring accessibility. Additionally, avoid using an overly vibrant palette that might distract from the data itself. A general rule is to use a neutral base and employ brighter hues sparingly for emphasis.
Incorporate Interactivity
Interactive elements such as hover tooltips, clickable legend filters, and drill-down functionalities can greatly enhance user engagement. This is particularly valuable for product insights, where different stakeholders might want to explore various slices of the data. An interactive dashboard allows them to filter by product version, user cohort, or timeframe, enabling tailored insights without leaving the visualization interface.
However, interactivity should be implemented judiciously. Overloading a dashboard with too many interactive widgets can overwhelm users, making it challenging to find the insights they need. Balance is key: provide enough interactive features to empower exploration but keep the layout clean and intuitive.
5. Common Pitfalls to Avoid
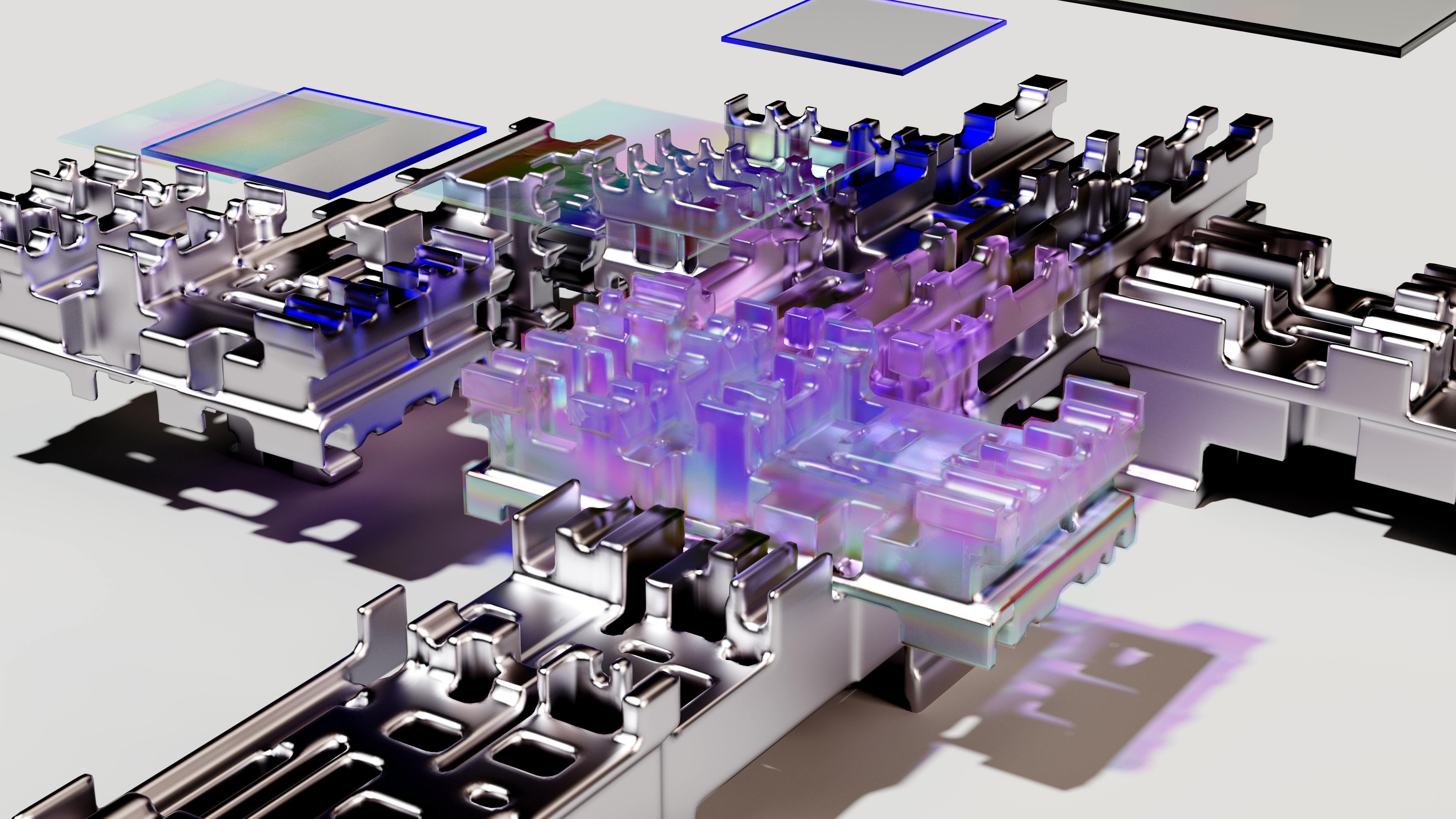
Overloading with Information
One of the most frequent mistakes in data visualization is attempting to include every piece of data in a single view. While it might be tempting to showcase how comprehensive your analysis is, excessive data can dilute the impact of the insights. The audience, faced with a wall of information, may struggle to discern the key points. Rather than layering multiple metrics on a single chart, consider creating separate views or using interactive filters that can reveal additional data on demand.
Overloading can also manifest in cluttered labeling and excessive annotations. Strive to keep annotations concise and ensure that each label contributes to understanding. If you find the labels and callouts overshadowing the chart’s main data points, consider alternative approaches like hover tooltips or a simplified legend system.
Misleading Visuals
Even well-intentioned visualizations can be misleading if you manipulate scales, truncate data, or selectively highlight certain ranges. For example, if your line chart’s y-axis doesn’t start at zero, a minor fluctuation in your data may appear exaggerated. Likewise, using pie charts with too many slices can make it difficult to compare each slice accurately. Ensuring that your visuals adhere to basic design and statistical principles is essential for maintaining credibility.
Another subtle form of misleading visuals arises when the color scale is not intuitive or is skewed to emphasize certain values over others. Always verify that the scale you choose accurately reflects the range of your data. When in doubt, run your visuals by colleagues or external reviewers to see if they interpret the data in the manner you intend.
Ignoring Context
A beautiful, clear chart can still fall short if the audience lacks the context to interpret it. Context could mean historical data for comparison, industry benchmarks, or an explanation of key events that influenced metrics. For instance, if you notice a spike in user sign-ups in March, you should clarify whether this corresponds to a new product launch or a marketing campaign. Without this contextual understanding, viewers might jump to inaccurate conclusions.
Always accompany your visuals with brief descriptions or supplemental information that explains why certain numbers matter. A well-placed text box highlighting significant events or milestones can guide the audience in connecting the data to real-world occurrences. Providing this additional layer of insight enhances the credibility and usefulness of your presentation, ensuring that viewers come away with both facts and the story behind those facts.
7. Future Trends in Data Visualization
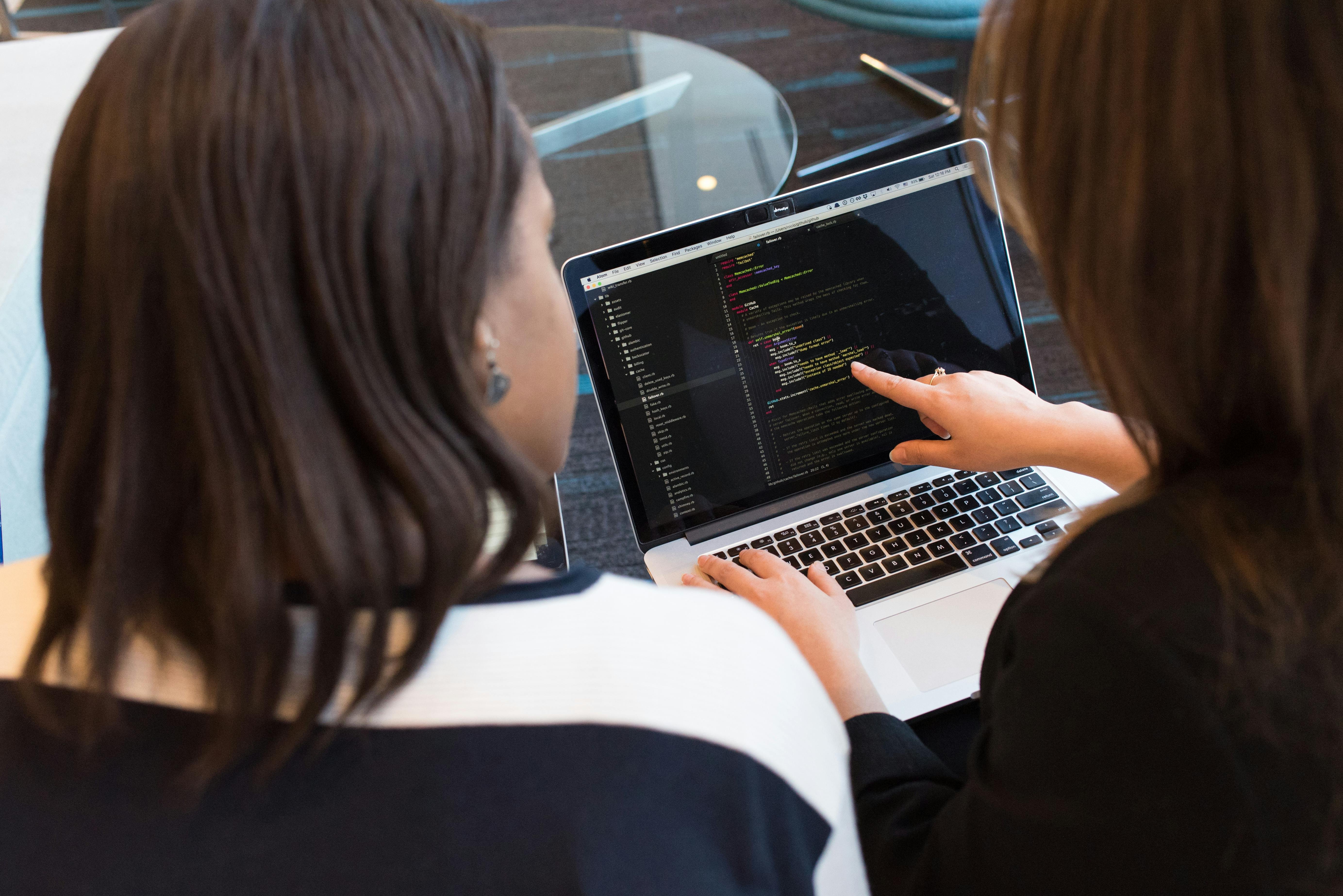
The field of data visualization is continuously evolving, driven by advancements in technology and the ever-growing appetite for real-time, actionable insights. In the context of product analytics, staying abreast of emerging trends can offer a competitive edge. Let’s examine some of the shifts and innovations that promise to reshape how we visualize and interpret product data.
Emerging Technologies: AI and VR
Artificial Intelligence (AI) is making its mark on data visualization through automated chart suggestions, anomaly detection, and predictive analytics. Tools powered by machine learning algorithms can analyze data sets and recommend the most appropriate visualization types based on data patterns. This takes much of the guesswork out of the design process, allowing organizations to rapidly iterate through potential visuals to find the one that best illuminates a business question.
Virtual Reality (VR) and Augmented Reality (AR) are also on the horizon for data exploration. These technologies have the potential to create immersive data experiences, enabling stakeholders to navigate through complex 3D data environments. Imagine a product manager literally walking through a map of user engagement metrics, where peaks and valleys correspond to areas of high and low activity. While still nascent, VR and AR can unlock new ways of perceiving multidimensional data that traditional screens might struggle to convey.
Personalization and Advanced Analytics
Another growing trend is personalized dashboards and analytics. As data repositories expand, it becomes impractical to show every stakeholder the same universal dashboard. Instead, advanced tools allow for dynamic personalization, so product managers see visualizations tailored to their modules, while marketing teams see campaign-specific metrics. This approach streamlines decision-making by ensuring each role has immediate access to the most relevant insights.
Meanwhile, the integration of advanced analytics methods such as predictive modeling and text analytics is transforming how product insights are derived. Beyond descriptive analytics, organizations are turning to machine learning models to predict user churn or identify the best time to launch a new feature. Visualizing these predictive models and their confidence intervals empowers teams to make proactive decisions rather than merely reacting to historical data.
Trends in Product Insights
The nature of product insights themselves is evolving. With the explosion of IoT devices and edge computing, more data streams are generated closer to the user—think wearable devices, smart home appliances, or intelligent vehicles. Visualization strategies are adapting to handle these streaming data sources, placing greater emphasis on real-time dashboards. Instead of waiting for end-of-day or weekly reports, stakeholders can watch metrics unfold in near real-time and make rapid adjustments.
Additionally, an increased focus on user privacy and compliance is altering how data is collected and visualized. Stricter regulations on data collection require that organizations adopt careful approaches to data anonymization and aggregation. In many cases, this involves presenting product usage metrics in aggregated forms to protect individual user identities. Future dashboards will likely feature more sophisticated ways of anonymizing data while still delivering actionable insights, requiring data visualization designers to adapt their methods accordingly.
Conclusion
Throughout this article, we’ve traversed the landscape of data visualization as it pertains to effectively presenting product insights. We began with the foundational understanding of what data visualization is and why it’s indispensable for modern businesses. We then explored the key principles—clarity, simplicity, accuracy, and consistency—that underpin compelling visuals. From choosing the right tool to designing audience-specific dashboards, each step is integral to ensuring your visualizations don’t just look good but also facilitate informed decision-making.
We also covered common pitfalls like overloading information, misleading visuals, and neglecting context—mistakes that can quickly erode the trustworthiness of your data and stall the momentum of data-driven initiatives. Through real-world case studies, we saw how carefully crafted visual analytics can expose critical insights into user behavior, feature adoption, and product performance. Finally, by looking at future trends—ranging from AI-driven automation and VR experiences to personalized dashboards and ethical data collection—we recognized that data visualization is a dynamic field that continues to expand its reach.
Mastering data visualization is more than just learning a new tool or skill—it’s about cultivating a mindset that seeks clarity and narrative in data. In the fast-paced environment of product development, where each insight can shape the direction and success of a launch, powerful and transparent data visualization can serve as a lynchpin for cross-functional collaboration. By adhering to best practices, staying updated on emerging technologies, and focusing on what truly matters to your audience, you can transform raw data into actionable strategies that resonate across your organization.
As a next step, we encourage you to apply these best practices in your own work. Start with a clear objective, choose the appropriate visualization tool and chart types, and continue iterating based on feedback. For more tips, resources, and in-depth guides, explore the additional materials available on this site. Whether you’re looking to refine your existing dashboards or embark on a new visualization project, the principles and insights covered here will lay a solid foundation for success. Embrace the art and science of data visualization, and watch how it can drive impactful, data-driven decisions that propel your product and business forward.
What next?
Take a look at more of our resources to learn more about Data Analytics.