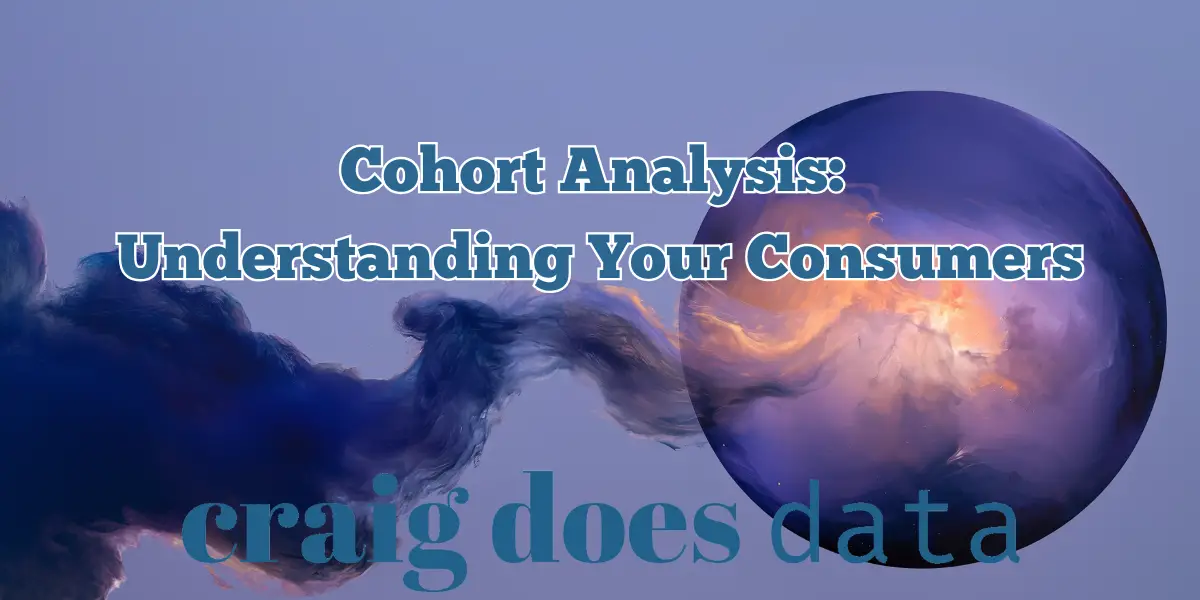
Cohort Analysis: Understanding Your Consumers
Businesses today operate in highly competitive environments, where winning consumer attention and loyalty is no small feat. Success often depends on how well you can understand—and ultimately cater to—the specific needs and behaviors of your audience. Imagine having the ability to pinpoint when customers first discover your brand, how they behave during each stage of the customer lifecycle, and what triggers them to continue (or discontinue) their engagement with your products or services. That is precisely the kind of insight that cohort analysis offers.
Cohort analysis is not merely another buzzword in analytics circles—it is a powerful method that enables you to observe changes in user behavior over time, grouped by shared characteristics or experiences. Rather than looking at a broad, faceless mass of users, you break your audience down into smaller, more manageable groups (cohorts). Through this lens, you can track performance, spot trends, measure retention, and unearth patterns that often go unnoticed in aggregate data reports.
In this article, we will explore the ins and outs of cohort analysis, explaining how it differs from other data analytics methods and why it has become an essential tool for businesses in the digital age. If you have ever struggled to figure out why certain marketing campaigns drive conversions more effectively than others, or if you wonder how to keep your customers coming back, then you are in the right place. Cohort analysis can drastically improve how you approach customer engagement, retention, and long-term growth strategies. Read on to discover its intricacies, step-by-step processes, and real-world applications that you can start implementing today.
What is Cohort Analysis?
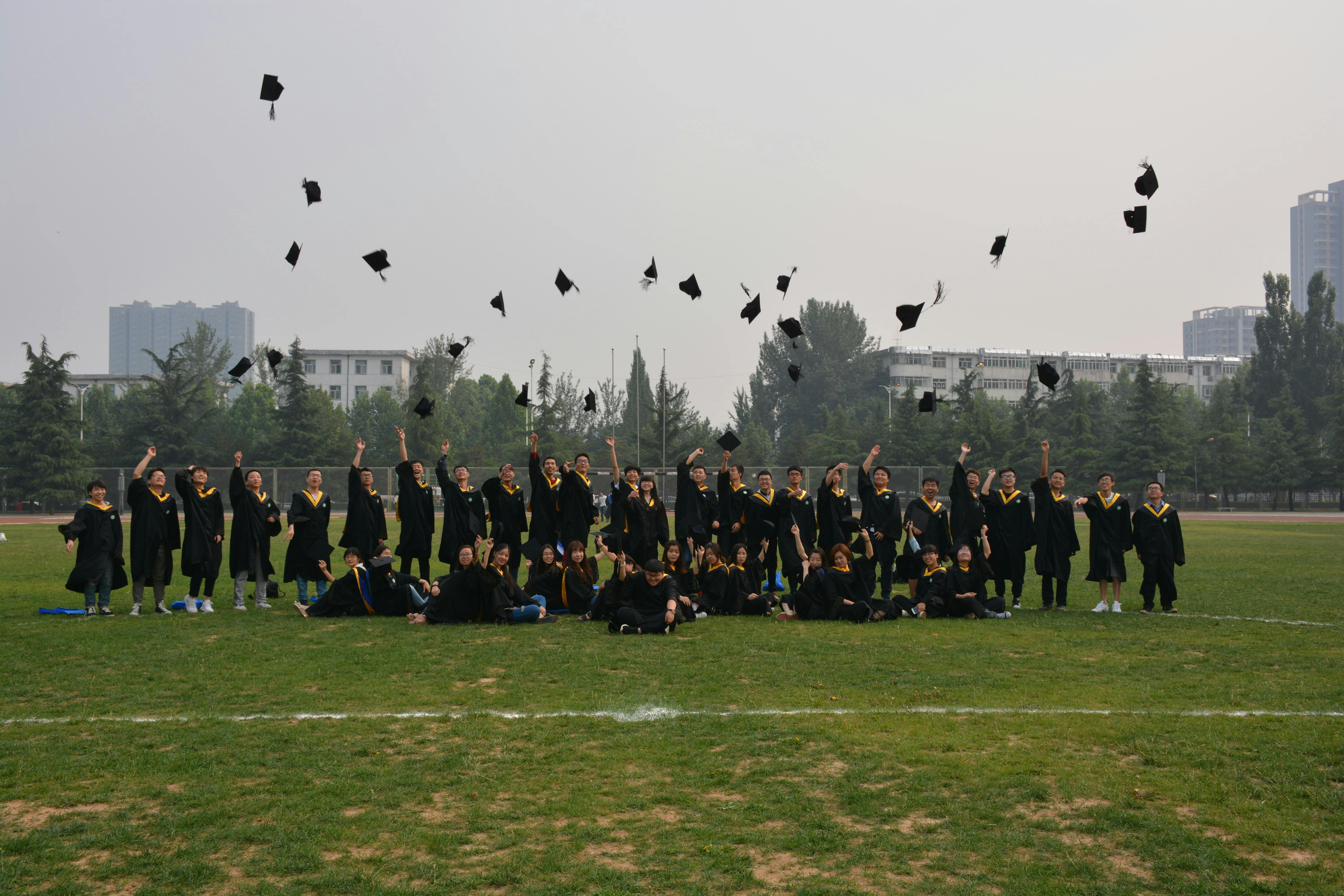
At its core, cohort analysis is a type of behavioral analytics that groups users based on shared characteristics, typically within a defined time range. For instance, a cohort might consist of all customers who made their first purchase during a specific month or all visitors who signed up for a subscription on the same day. Rather than analyzing the entire customer base as one homogeneous entity, the concept of a cohort allows you to study how these users behave collectively from the point of their first interaction or based on the behavior they exhibited.
One might wonder how this differs from traditional segmentation or other trend analyses. In segmentation, you might categorize your users based on demographic or psychographic attributes—such as age, location, or interests—without necessarily linking their behaviors to a specific starting point in time. Traditional trend analysis might look at overall sales or engagement metrics over a certain period, but it usually fails to isolate the nuanced behaviors of different user groups who began their journey at different times or in different ways.
Cohort analysis is unique because it weaves in the temporal element, thereby allowing you to see how certain groups evolve over their lifespan with your brand. If you see, for example, that your acquisition cohorts from January and February behave very differently than those from March or April, you gain insight into how external factors—like seasonality, changes to product offerings, or variations in marketing campaigns—impact long-term engagement. This type of granular, time-based segmentation offers a deeper perspective that can be crucial for crafting effective customer retention strategies, optimizing marketing spend, and steering product development efforts.
The Importance of Cohort Analysis in Understanding Consumers
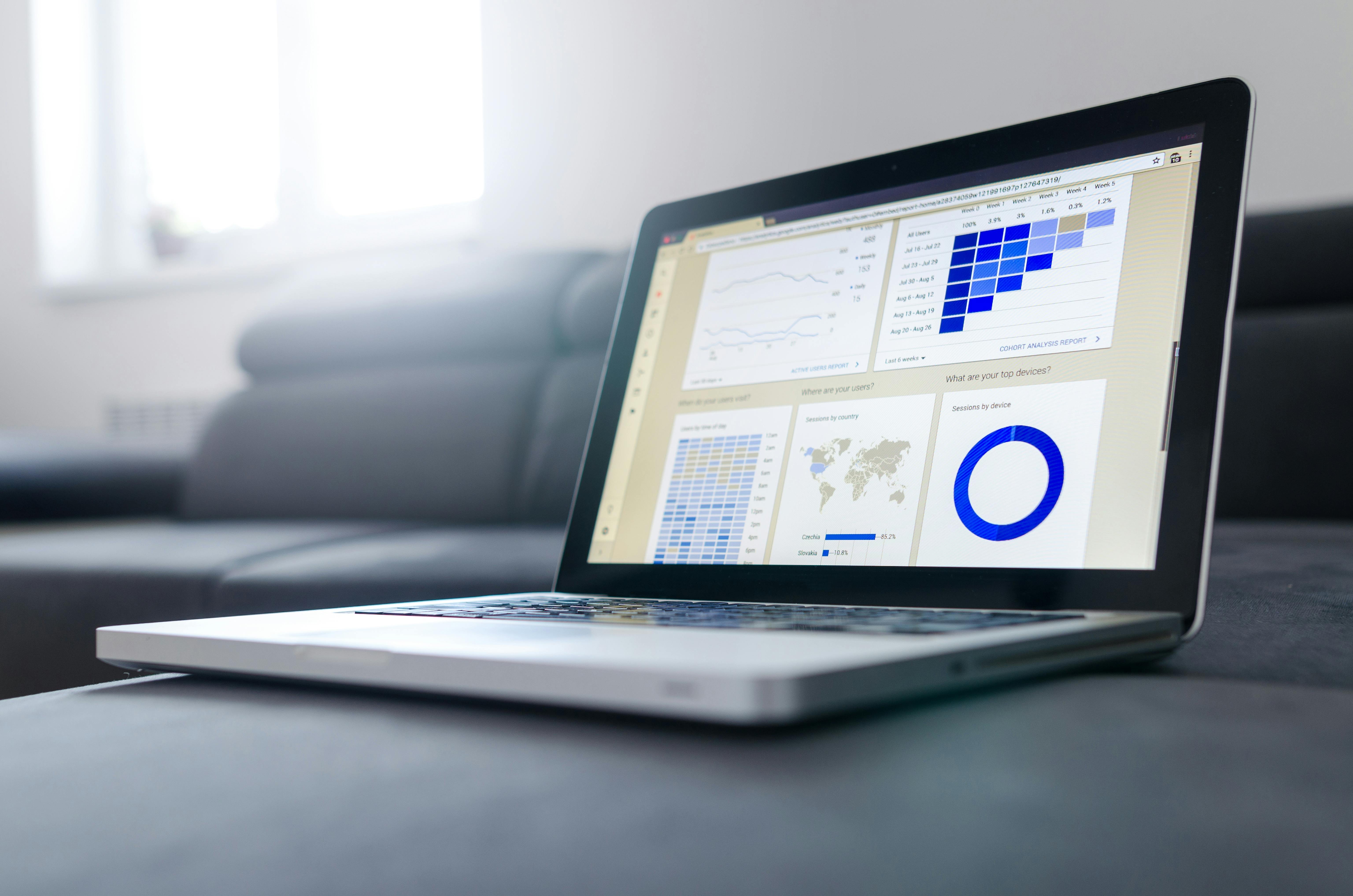
Consumers are the lifeblood of any business, and understanding their journeys from the moment they discover a brand to the point they become advocates (or churn) is paramount. Here is how cohort analysis shines in various dimensions:
- Deeper Consumer Insights: By focusing on a set of users who share a common event or characteristic, you can track how their behavior changes over time. This reveals patterns such as an increase in engagement after a particular product update or the point at which many of them start to lose interest. Such granular knowledge can guide product roadmap decisions and marketing approaches.
- Lifetime Value Calculation: Calculating Lifetime Value (LTV) is often guesswork without a clear view of how users behave after their initial purchase. Cohort analysis ties user behavior to their start date or key action date, making it much easier to forecast the revenue a particular cohort will generate over its lifetime. This, in turn, influences budget allocations, marketing spending, and overall strategy.
- Retention Strategies: Retention is often more cost-effective than acquisition. By applying cohort analysis, you can identify when and why certain cohorts drop off, thereby adjusting your strategies to intervene before the drop in engagement becomes a churn. You might discover, for instance, that users who do not complete a tutorial or who fail to utilize a particular feature early on are more likely to abandon the platform. These findings help shape onboarding processes, customer support resources, and feature updates.
In essence, cohort analysis enables you to see consumers not just as singular data points in a dashboard, but as dynamic groups whose behavior evolves under various influences. It helps you learn which initiatives truly resonate with customers over time, providing a laser-focused approach to making informed decisions that bolster growth, loyalty, and profitability.
How to Perform Cohort Analysis
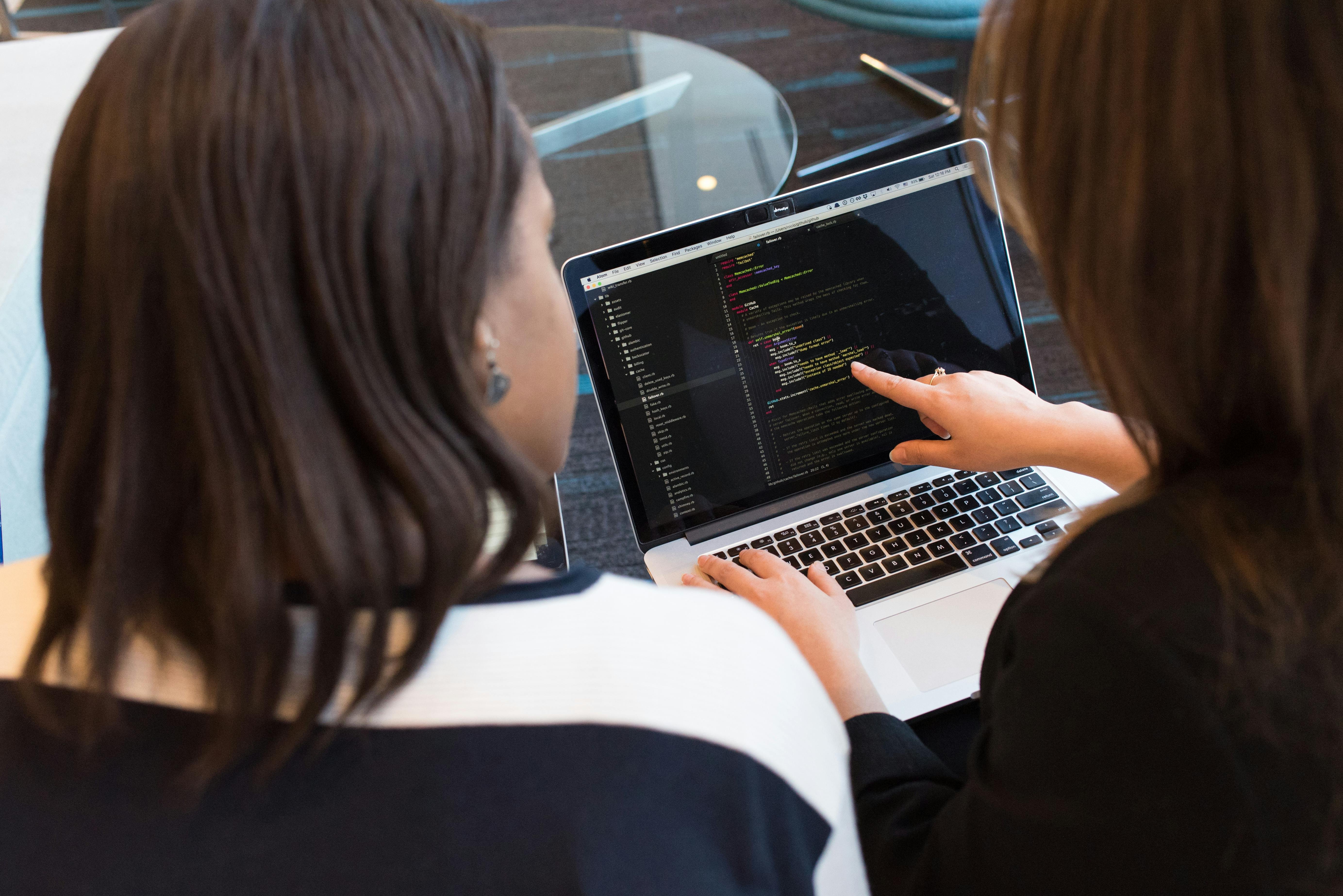
While the idea behind cohort analysis is straightforward, performing it effectively requires a structured approach and careful consideration of data hygiene, tools, and metrics. Below is a step-by-step guide to help you navigate the process:
- Define Your Objective: Before diving into data, clarify why you are conducting a cohort analysis. Are you looking to measure onboarding effectiveness? Do you aim to understand churn rates? The objective guides your selection of cohorts and metrics.
- Identify Your Cohorts: Decide on the type of cohorts you want to analyze (acquisition, behavioral, or retention cohorts). For instance, if your goal is to assess how marketing campaigns affect user acquisition, group users by the month they joined. If you want to analyze how a new feature influences engagement, form a behavioral cohort around the date they started using that feature.
- Collect and Clean Your Data: Ensure the data you rely on is accurate, timely, and relevant. This often involves integrating various data sources—CRM systems, analytics platforms, marketing automation tools—and cleaning the data to remove duplicates or fill in missing fields. Inaccurate data can skew results and lead to flawed conclusions.
- Choose the Right Metrics: Metrics may vary depending on your goal. Common metrics include conversion rate, churn rate, retention rate, average purchase value, or usage frequency. For instance, if you are analyzing retention over multiple months, you might track the percentage of users in each cohort who remain active in the following months.
- Analyze Trends and Patterns: Once your data is grouped, you can create visualizations like retention charts or pivot tables that make it easy to spot trends. Look for commonalities, anomalies, or spikes and dips in user engagement across different cohorts and time periods.
- Interpret and Implement Insights: The final step is turning data into action. If you notice a particular cohort has significantly lower retention past week four, investigate why. Is it linked to a product shortcoming? Did they have less engagement with your marketing emails? Use these insights to implement changes—whether in product design, customer support, or marketing strategies—to address the issues you uncover.
Tools and Software: Thankfully, you do not need to build everything from scratch. Multiple platforms facilitate cohort analysis. Google Analytics offers basic cohort exploration features, allowing you to group users by acquisition date and then track their behavior. Mixpanel is also popular for product analytics, offering a robust interface for creating and studying behavioral cohorts. For more advanced analyses, Amplitude provides detailed retention grids, funnels, and behavioral insights. If you prefer a more hands-on approach, you can use Python or R with libraries like pandas or dplyr for custom analyses, though this typically requires more technical expertise.
A well-executed cohort analysis can transform raw data into a compelling narrative about how your users interact with your brand over time. Armed with these insights, you can pivot strategies, invest in successful initiatives, and optimize user journeys to ensure that customers remain engaged and satisfied in the long run.
Types of Cohorts in Analysis
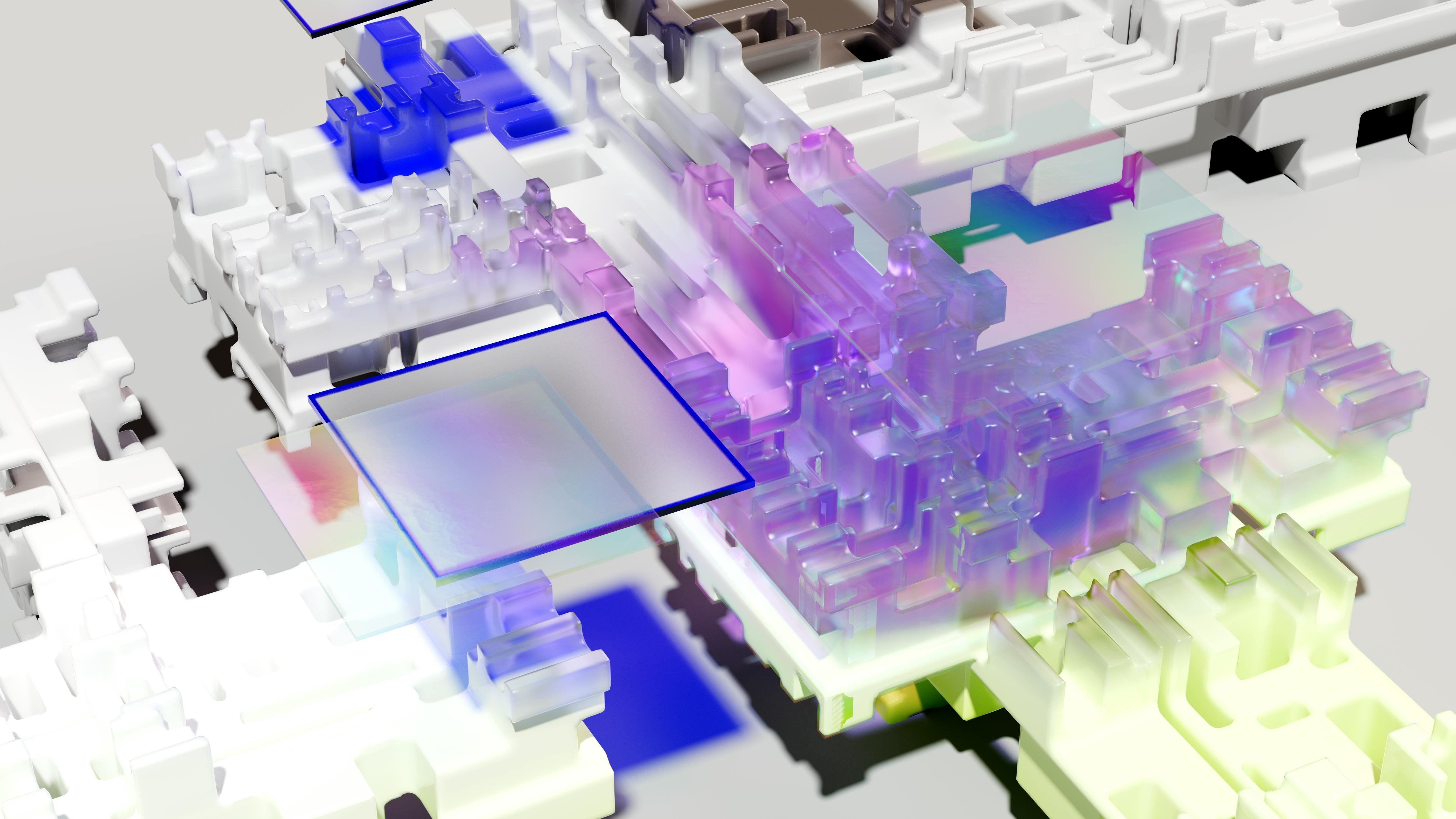
While cohort analysis fundamentally hinges on grouping users with common traits or starting points, there are different ways to slice this concept. Each type of cohort focuses on a specific aspect of user behavior or engagement timeline:
Acquisition Cohorts
Acquisition cohorts group users based on the time they first interacted with your business—this could be the day they signed up for your newsletter, downloaded your app, or made their initial purchase. Analyzing these cohorts allows you to measure how users who joined in one period differ in engagement from those who joined in another. You might discover that users who signed up in December have a higher average purchase value than those who signed up in April, potentially indicating seasonality or differences in promotional campaigns. Such insights can guide future acquisition strategies and resource allocation.
Behavioral Cohorts
Behavioral cohorts group users by their actions or inactions. Perhaps you want to observe how users who utilized a specific feature—like a shopping cart reminder—behave over time compared to users who never used that feature. This type of cohort analysis can highlight whether certain behaviors are linked to higher retention, more frequent purchases, or increased customer satisfaction. For instance, if you run a music streaming service, you might create a cohort of users who created at least one playlist in their first month and compare their churn rates to users who did not create any playlist. If the former shows significantly better retention, you have a clue that nudging new users to create playlists early on can boost overall engagement.
Retention Cohorts
Retention cohorts focus on how long users stay active or continue engaging with your brand. Often, this involves tracking the number or percentage of users from each cohort who remain active after a certain number of days, weeks, or months. If you notice retention dropping sharply after the second month, you might need to investigate common friction points or re-engagement strategies to keep users active. This type of analysis is especially popular in subscription-based and SaaS businesses, where monthly or annual renewal rates can make or break revenue projections.
Each of these cohort types serves a distinct purpose, but they are far from mutually exclusive. In fact, it is common for businesses to run multiple cohort analyses simultaneously, examining how acquisition and behavioral factors intersect to affect retention. By layering insights from different angles, you get a multifaceted understanding of your audience—one that guides more holistic decisions about marketing, product design, and customer success.
Real-World Applications of Cohort Analysis
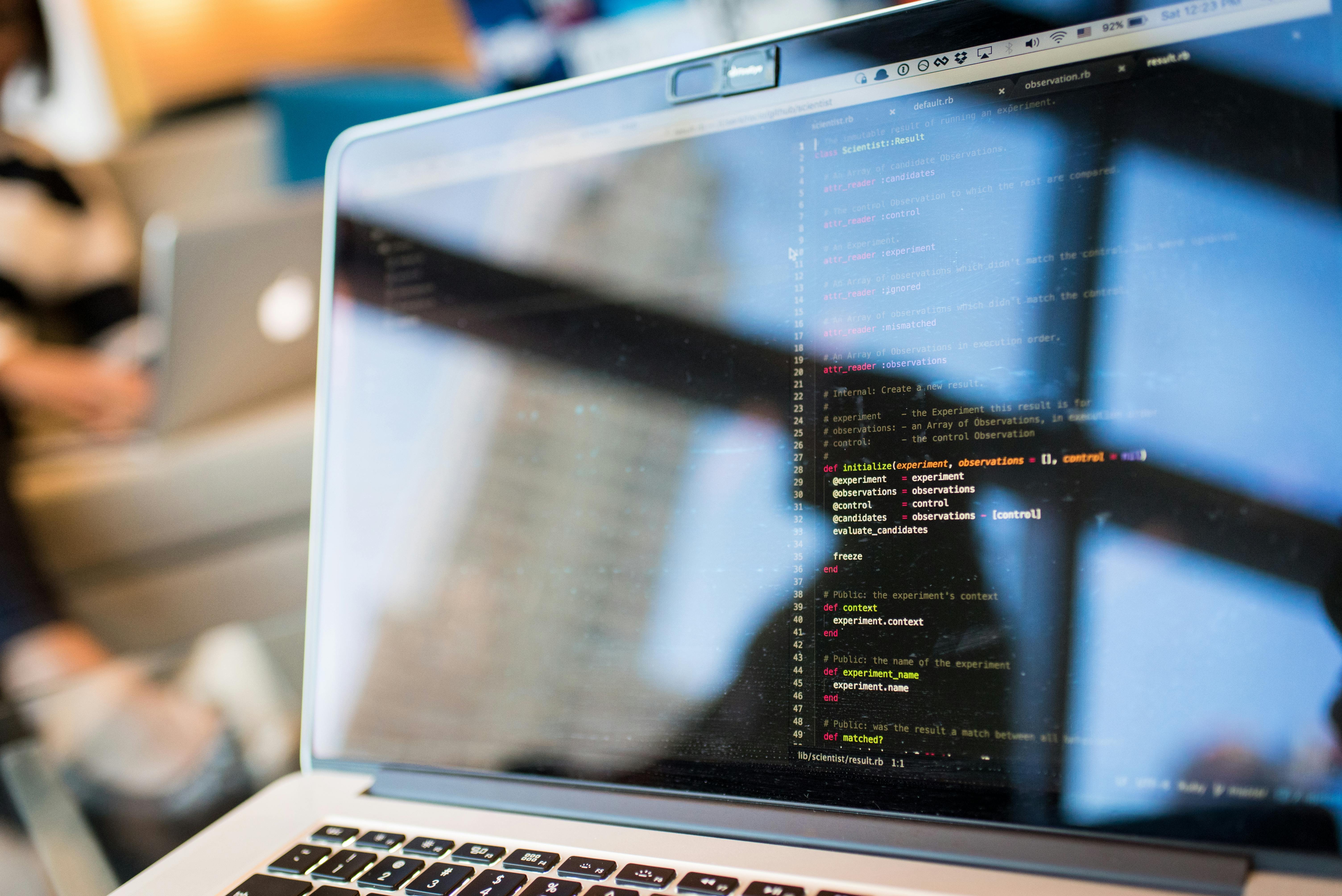
Cohort analysis is not just a theoretical exercise or an interesting dashboard metric; it is a practical, outcome-driven approach that has been used effectively across various industries. Here are some notable scenarios:
Case Study 1: E-Commerce Retention Strategies
An online clothing retailer noticed that while they were acquiring new customers steadily, their repeat purchase rate was dropping. By using acquisition cohorts, they identified that customers who initially purchased during a site-wide sale had a significantly lower return rate than those who purchased at full price. This insight suggested that sales-driven cohorts might be less brand-loyal or were primarily price-sensitive.
In response, the retailer developed targeted email marketing campaigns and loyalty points specifically for these price-sensitive cohorts. Over the next quarter, they saw a notable uptick in second and third purchases from these users, ultimately increasing overall retention by 15%. This case exemplifies how cohort analysis can guide tailored interventions to tackle segment-specific challenges.
Case Study 2: SaaS Onboarding Optimization
A SaaS company providing project management tools wanted to better understand why many of their trial users dropped off before converting to paid customers. By analyzing the onboarding behaviors of new sign-ups in monthly behavioral cohorts, they discovered a strong correlation between users who completed a guided product tour and those who became paid subscribers.
Their immediate response was to nudge new users more aggressively toward completing the product tour, implementing in-app reminders and email follow-ups. In the following two months, the conversion rate to paid subscriptions in these cohorts rose by 30%. Cohort analysis thus guided a precise product and marketing tweak that paid dividends in user retention and revenue growth.
Case Study 3: Mobile App Engagement
A mobile gaming company frequently introduces new levels and seasonal events. However, they were unsure which updates truly fostered long-term engagement and which were merely short-term spikes. Through a combination of behavioral and retention cohort analyses, the company noticed that users who participated in seasonal events had a significantly higher Day 30 retention rate compared to those who did not engage with the special content.
By doubling down on thematic events, offering unique in-game rewards, and refining the event mechanics, the game saw a sustained increase in user retention. This validated the importance of dynamic, regularly updated content to maintain player interest, highlighting the tangible business value that well-executed cohort analysis can deliver.
Whether it is e-commerce, SaaS, mobile applications, or any other sector that relies on recurring user engagement, cohort analysis offers actionable insights. It unravels hidden patterns that aggregate metrics might mask, enabling targeted interventions that align closely with user behavior. Over time, the improvements driven by these insights can significantly impact revenue, brand perception, and competitive advantage.
Challenges and Limitations of Cohort Analysis
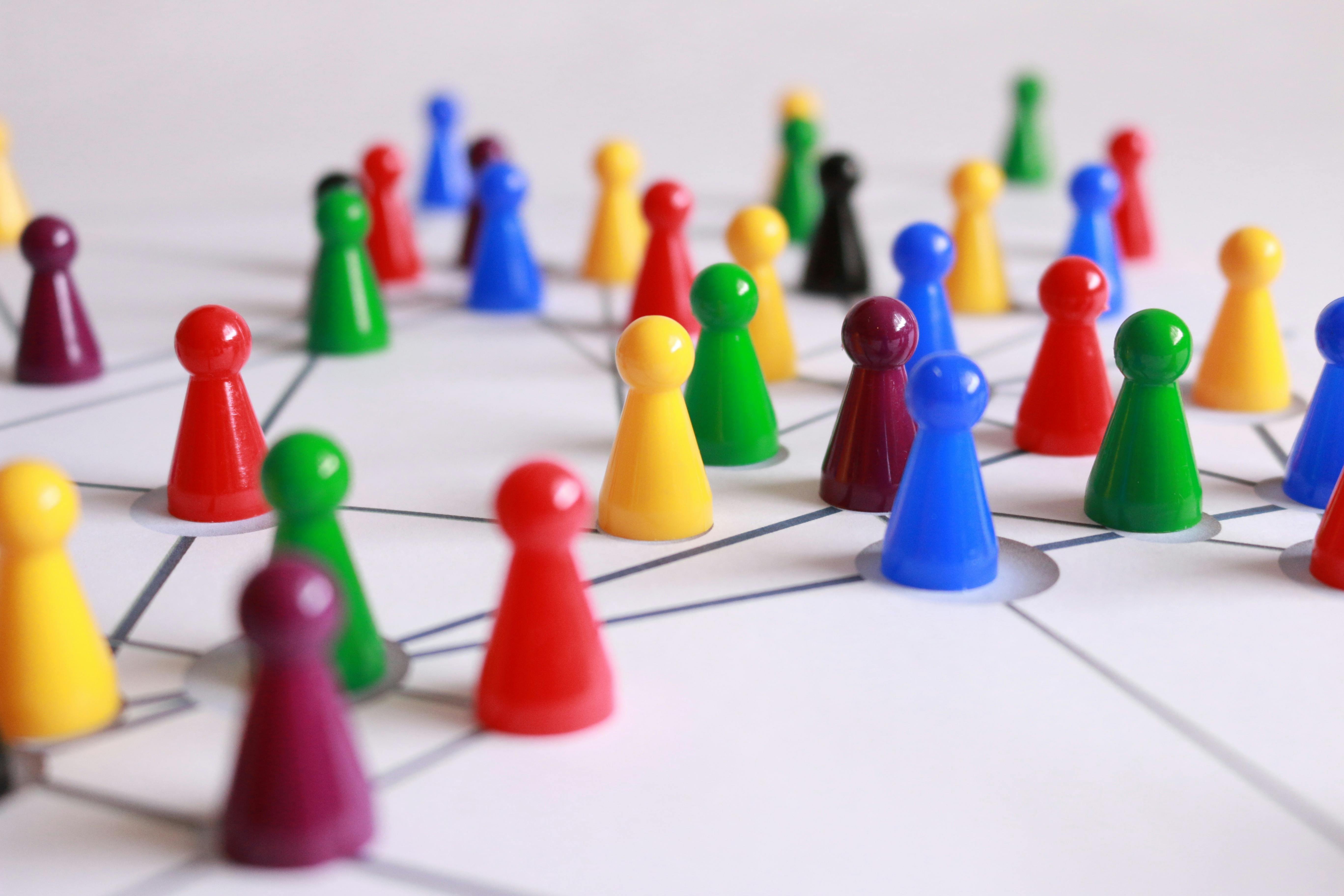
Despite its numerous benefits, cohort analysis is not without its difficulties. Understanding these pitfalls will help you plan more effectively and avoid misleading conclusions:
- Data Quality: If the underlying data is incomplete, inaccurate, or outdated, any cohort analysis derived from it will be flawed. Data collection systems should be robust and consistent. Minor data entry errors, duplicates, or unsynchronized databases can introduce bias in your cohorts.
- Complexity: For businesses with multiple products, channels, or user touchpoints, setting up a coherent cohort analysis can be complicated. Each new dimension (such as location, device type, or marketing channel) adds layers of complexity. Beginners may feel overwhelmed if they try to track too many variables at once.
- Dynamic Markets: Market conditions can shift rapidly. If your industry experiences frequent disruptions—be it new regulations, technological innovations, or macroeconomic changes—the behaviors you observe in older cohorts may not generalize to newer cohorts. You should interpret your results in the context of external factors and continually update your analyses to account for changing conditions.
- Interpretation Bias: Even when the data is accurate, the risk of misinterpretation looms. Correlation does not necessarily mean causation. Seeing that a particular cohort has lower retention may prompt assumptions that a specific event caused it. However, multiple factors—from seasonality to changes in competitor actions—could be at play.
While these challenges should not discourage you from employing cohort analysis, they underline the necessity of meticulous planning and cautious interpretation. Equip yourself with robust data management processes, maintain an awareness of evolving market conditions, and use complementary analytics methods (like A/B testing or user interviews) to validate or contextualize your findings.
Best Practices for Cohort Analysis

To harness the full potential of cohort analysis, it is wise to follow tried-and-true best practices. These ensure that you not only generate reliable insights but can also act on them effectively:
- Consistent Tracking: Establish unified standards for data collection across different teams and platforms. Make sure the events you track—such as sign-ups, purchases, or app launches—are clearly defined and consistently captured. Consistency ensures that cohort definitions remain comparable over time.
- Regular Updates: Markets change, campaigns shift, and product features evolve. A cohort analysis should be a living process rather than a one-off project. Schedule regular updates—weekly, monthly, or quarterly—to reassess and realign your findings with current realities.
- Segmentation Within Cohorts: One way to dig deeper is to segment cohorts by other attributes like location, user intent, or referral source. This extra layer can expose nuanced behaviors and identify pockets of users who may require specialized strategies.
- Actionable Insights: Data alone is useless without interpretation and action. Always conclude your analyses by asking: “What can we do about this?” If you find a cohort that exhibits exceptionally high churn at a specific time, brainstorm and implement targeted interventions—like an in-app message, a personalized email, or a loyalty discount—to address the issue.
- Combine Qualitative Methods: Quantitative data can show you the what but not always the why. Supplement your cohort analysis with user interviews, surveys, or usability studies to understand the root causes behind observed behaviors.
Executing cohort analysis effectively is less about the complexity of the tool you use and more about the quality of your methodology and your willingness to take decisive action based on what the data reveals. By maintaining rigorous standards for data collection, regularly reviewing cohorts, and coupling findings with real-world interventions, you maximize the impact of your analysis on overall business performance.
Conclusion
Cohort analysis is an indispensable technique for businesses eager to understand not just how many users they have, but how and why those users choose to engage or disengage over time. By breaking your audience into smaller, more coherent groups based on shared characteristics or behaviors, you gain the ability to spot trends and patterns hidden in aggregate data. Whether you are an e-commerce store aiming to improve repeat purchases, a SaaS platform refining your onboarding process, or a mobile gaming company looking to enhance engagement, cohort analysis provides the lens through which you can see the journey of your customers more clearly.
The real value of cohort analysis lies in its capacity to drive action. Armed with these time-based insights, you can refine your product roadmap, tailor your marketing campaigns, and build meaningful retention strategies. While it does require robust data and careful interpretation, the potential gains—from boosting Customer Lifetime Value to driving sustainable, long-term growth—can be transformative.
As consumer behavior continues to evolve in an increasingly digital and competitive marketplace, the ability to perform cohort analysis effectively will become an ever-more critical component of data-driven decision making. Now is the time to harness its power and keep your business on the leading edge of consumer understanding and engagement.
Additional Resources
If you are eager to explore cohort analysis in even greater depth, here are some valuable resources that can help you continue your learning journey:
-
Books and Articles:
- Lean Analytics by Alistair Croll and Benjamin Yoskovitz – Offers frameworks for measuring business success and includes discussions on cohort analysis.
- Online articles on Medium and Towards Data Science often feature case studies and how-to guides for different analytics topics, including cohort analysis.
-
Online Courses:
- Udemy and Coursera courses on business analytics or data science frequently include modules on cohort analysis.
- Mixpanel's own tutorials provide a comprehensive look at how to use its platform for cohort-based insights.
- Google Analytics Academy offers lessons on how to leverage built-in cohort features.