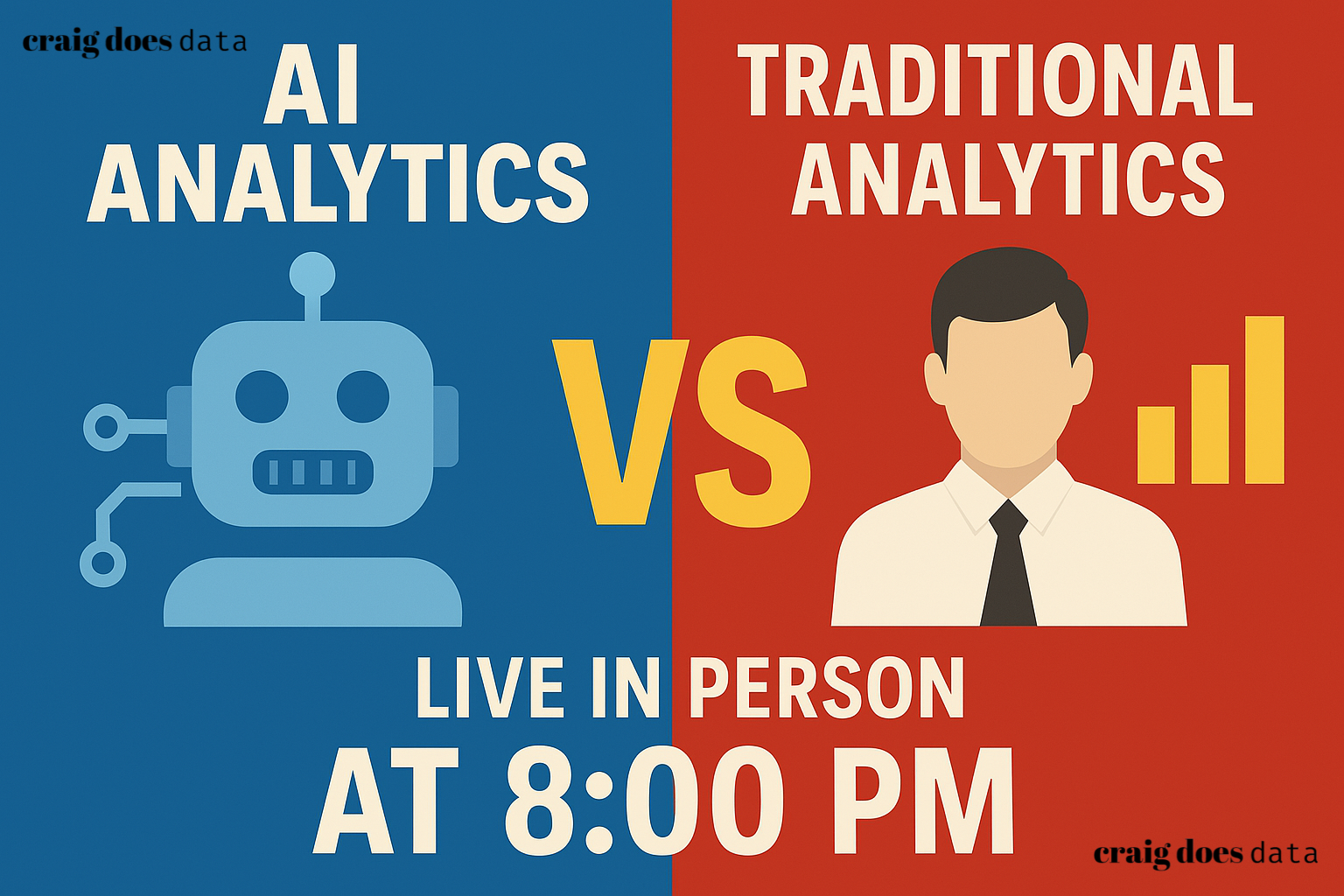
AI vs Traditional Analytics: The Strengths and Weaknesses of Each and How They Complement Each Other
In today's rapidly evolving business landscape, data analytics has surged to the forefront as a critical component for informed decision-making and strategic planning. Organizations across all sectors are increasingly recognizing the immense value hidden within their data, and the ability to effectively extract and interpret this data is becoming a key determinant of success. At the heart of this data revolution are two distinct yet interconnected approaches to analytics: AI-driven analytics and traditional analytics.
Traditional analytics, the established and time-tested methodologies, have long been the backbone of business intelligence. It encompasses techniques like descriptive statistics, regression analysis, and data visualization, focusing on understanding historical data and identifying trends. On the other hand, AI-driven analytics represents the cutting edge, leveraging the power of artificial intelligence, machine learning, and natural language processing to delve deeper into data, uncover complex patterns, and even predict future outcomes.
This comprehensive guide aims to provide a detailed exploration of both traditional and AI-driven analytics. We will dissect each approach, examining their respective strengths and weaknesses, and, crucially, demonstrate how they can work in harmony to provide a more holistic and powerful analytical capability. Our goal is to demystify these concepts and offer practical insights for business professionals, especially small business owners, who are looking to leverage data analytics to drive growth, efficiency, and innovation. Whether you are just starting your data journey or seeking to enhance your existing analytics strategy, this article will provide a clear roadmap to understanding and utilizing the combined power of AI and traditional analytics.
Understanding Traditional Analytics
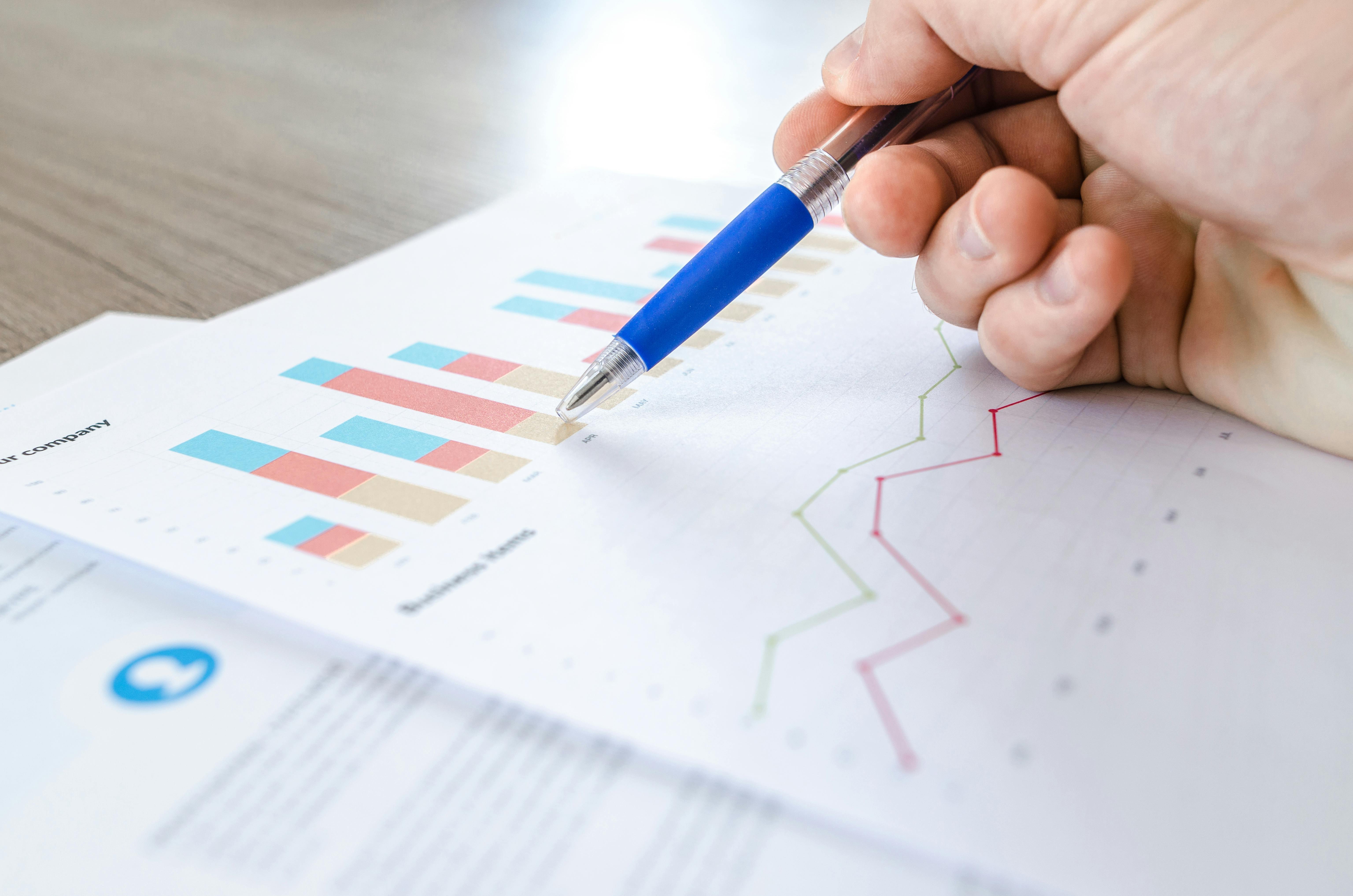
Traditional analytics forms the bedrock of data-driven decision-making, relying on established statistical methods and business intelligence techniques to interpret historical data. At its core, traditional analytics seeks to answer questions about what has happened and why, providing a clear picture of past performance and trends. This approach is fundamental for businesses to understand their current standing, identify areas of success, and pinpoint areas needing improvement. It’s about gaining clarity from the data you already possess, using proven methodologies that have been refined over decades.
The toolkit of traditional analytics is broad and well-defined. Descriptive statistics, for example, are used to summarize and present data in a meaningful way, using measures like mean, median, mode, and standard deviation. This helps in understanding the central tendencies and variability within datasets. Regression analysis is another cornerstone, used to model the relationship between variables. For instance, businesses might use regression to understand how marketing spend affects sales revenue. Data visualization techniques, including charts, graphs, and dashboards, play a crucial role in making complex data accessible and understandable to a wider audience, facilitating easier interpretation and communication of findings. Tools like Tableau, Power BI, and Qlik are commonly used to perform these operations and create insightful reports and visualizations. Spreadsheets, like Microsoft Excel and Google Sheets, also remain powerful and accessible tools for many traditional analytical tasks, particularly for smaller datasets and businesses.
Traditional analytics plays a crucial role in various business functions. In finance, it’s used for financial reporting, performance analysis, and risk assessment. Marketing teams leverage it to analyze campaign performance, understand customer segmentation, and measure ROI. Operations departments use traditional analytics for process optimization, quality control, and supply chain management. Sales teams rely on it for sales forecasting, pipeline analysis, and customer relationship management. The applications are virtually limitless, making traditional analytics an indispensable part of any organization seeking to operate efficiently and make informed decisions based on factual data.
Strengths of Traditional Analytics
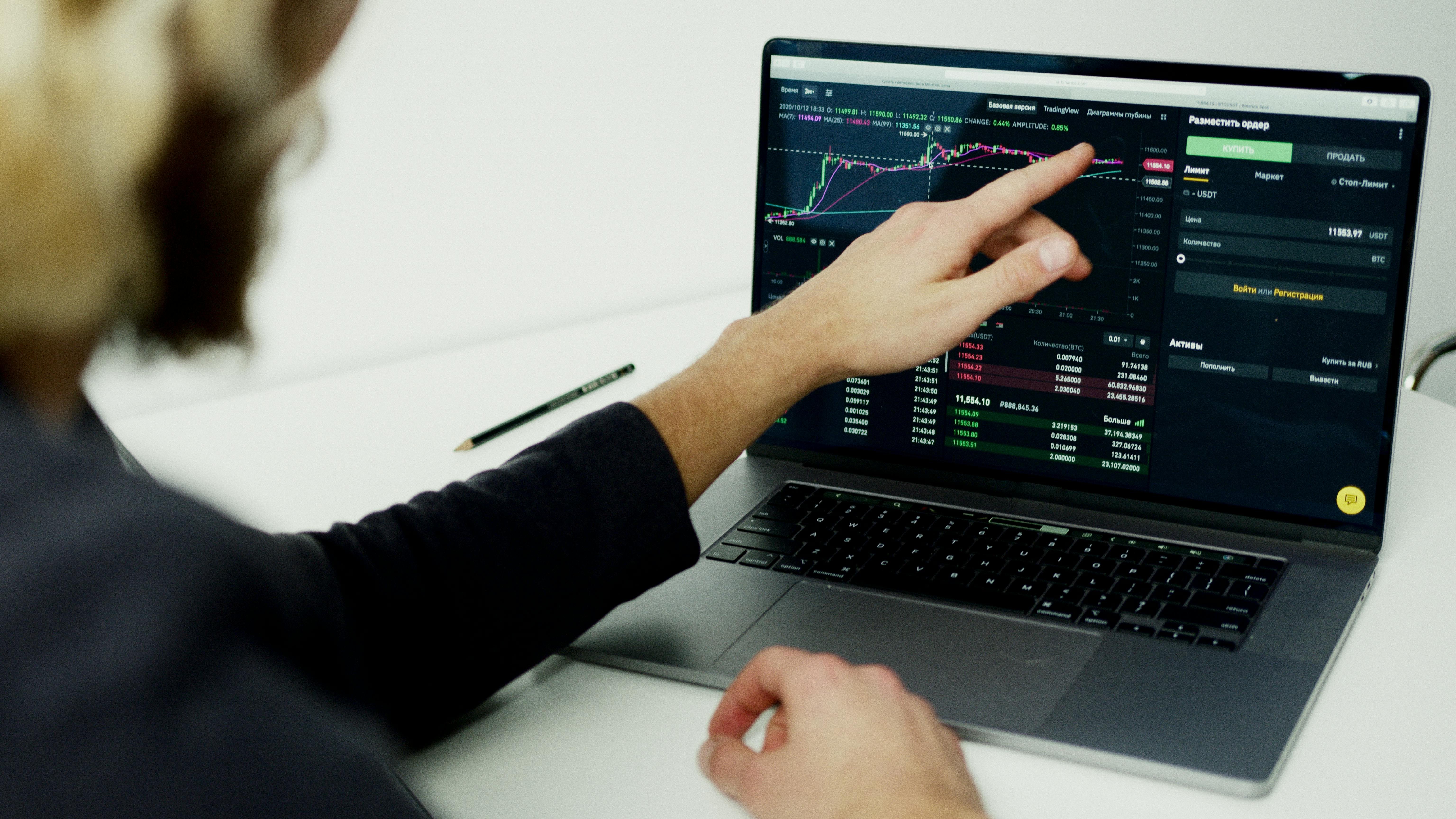
- Reliability and Established Methodologies: Traditional analytics benefits from decades of development and refinement. The methodologies used, such as statistical analysis and regression, are well-understood, rigorously tested, and widely accepted. This maturity ensures a high degree of reliability in the results, making it easier to trust the insights derived. Businesses can rely on these established methods knowing they are built on solid statistical and mathematical foundations. This reliability is particularly important in regulated industries or for critical business decisions where accuracy and verifiability are paramount.
- Simplicity and Interpretability: One of the key strengths of traditional analytics is its simplicity and ease of interpretation. Techniques like descriptive statistics and basic visualizations are straightforward to understand, even for individuals without a deep technical background in data science. The results are often presented in a clear and concise manner, making it easier for business users to grasp the key findings and their implications. This interpretability is crucial for effective communication of insights across different levels of an organization, ensuring that everyone can understand and act upon the analytical results.
- Low Cost and Ease of Implementation for Small Businesses: Traditional analytics tools and techniques are generally more affordable and easier to implement compared to AI-driven solutions. Many of the necessary tools, such as spreadsheet software and basic business intelligence platforms, are already part of standard business software suites. Furthermore, the skills required for traditional analytics are more widely available, and training resources are abundant and less specialized. For small businesses with limited budgets and technical expertise, traditional analytics offers a cost-effective and accessible entry point into data analysis, allowing them to gain valuable insights without significant investment in advanced technologies or specialized personnel.
Weaknesses of Traditional Analytics
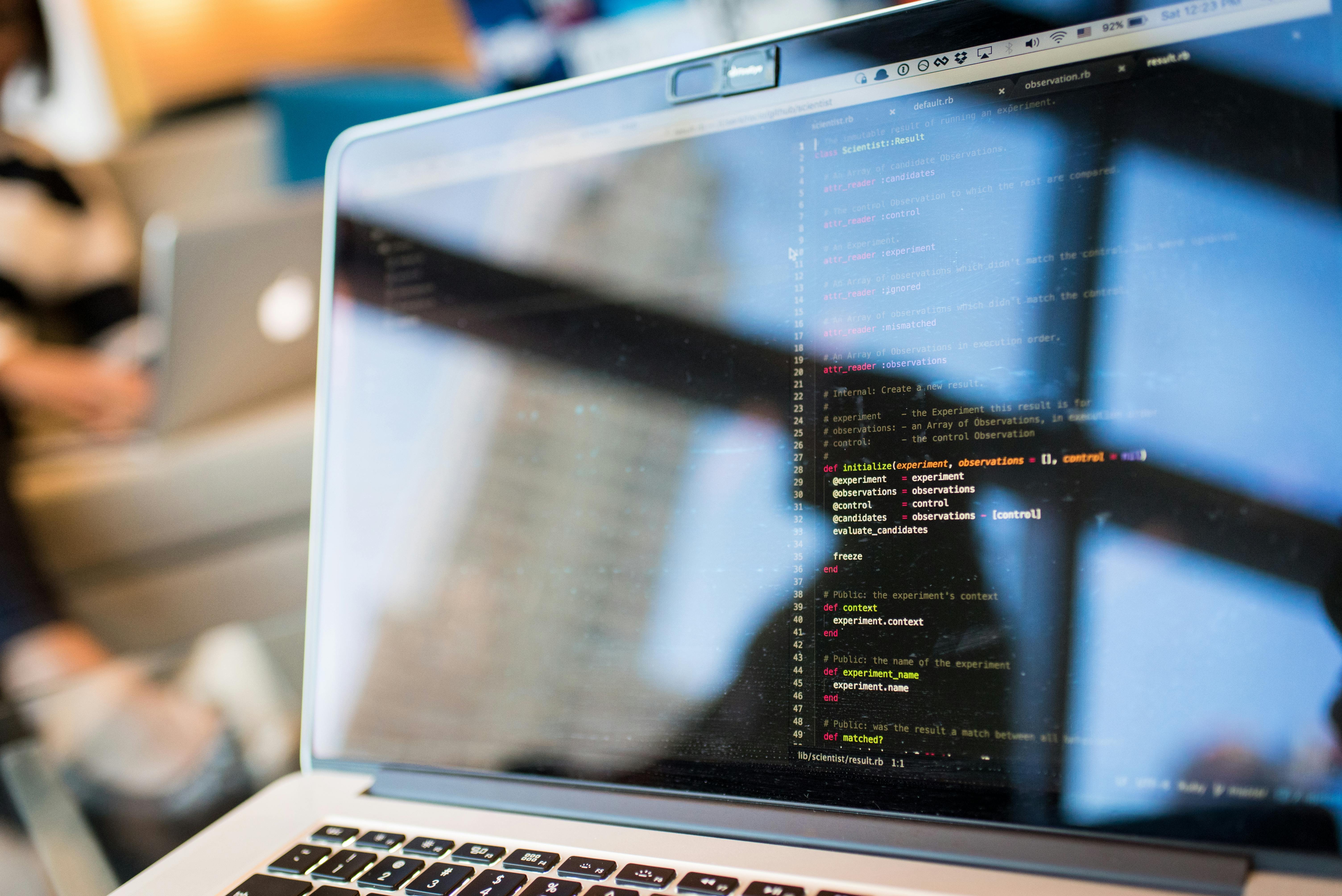
- Limitations in Handling Large Datasets: Traditional analytics methods can struggle when faced with the massive datasets characteristic of today’s big data environment. Techniques like manual data manipulation in spreadsheets or basic statistical analyses may become inefficient or even impossible to perform on datasets with millions or billions of data points. The computational resources and time required to process large datasets using traditional tools can become prohibitive, limiting the scope and depth of analysis. This limitation is increasingly significant as businesses generate and collect ever-larger volumes of data from various sources.
- Potential for Human Bias in Data Interpretation: While traditional analytics aims for objectivity, the interpretation of results can still be influenced by human bias. Analysts' preconceived notions, assumptions, or limited perspectives can inadvertently shape the way data is analyzed and the conclusions drawn. For example, in regression analysis, the selection of variables and the interpretation of coefficients can be subjective and influenced by the analyst's understanding or biases. This potential for bias can lead to skewed insights and potentially flawed decision-making.
- Challenges in Adapting to Real-Time Data Analysis Needs: Traditional analytics is often geared towards analyzing historical data in batch processing mode. Adapting to the demands of real-time data analysis, where insights are needed instantaneously, can be challenging. Traditional methods typically involve data extraction, transformation, and loading (ETL) processes, which are not designed for continuous, real-time data streams. While some traditional BI tools offer near real-time capabilities, they often lack the speed and agility required for truly real-time decision-making in dynamic environments. For businesses operating in fast-paced industries or requiring immediate responses to changing conditions, the limitations of traditional analytics in real-time processing can be a significant drawback.
Exploring AI-Driven Analytics
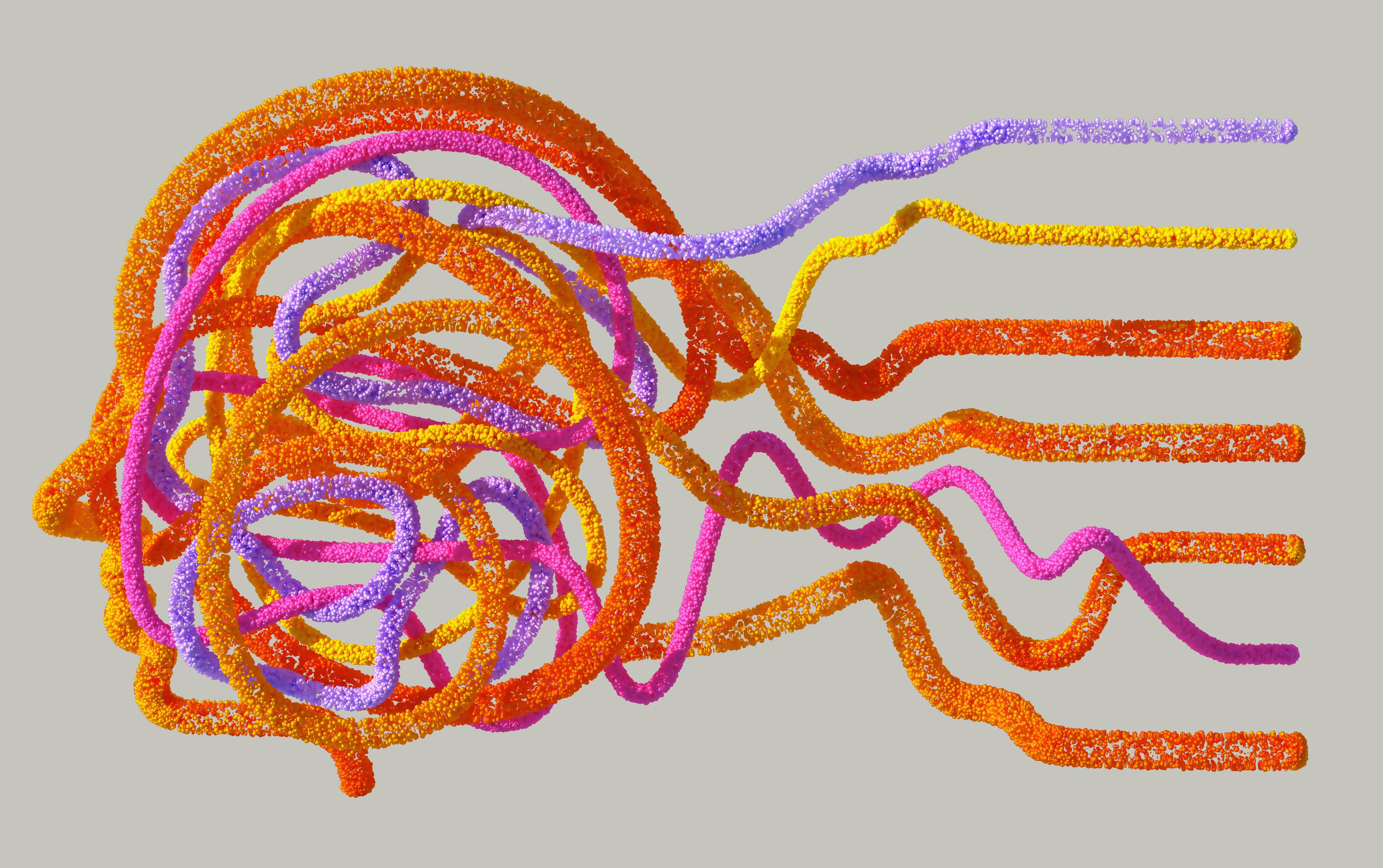
AI-driven analytics represents a paradigm shift in data analysis, moving beyond the descriptive and diagnostic capabilities of traditional methods to embrace predictive and prescriptive insights. It harnesses the power of artificial intelligence technologies, including machine learning, deep learning, natural language processing (NLP), and neural networks, to extract deeper, more complex, and more actionable intelligence from data. AI-driven analytics is not just about understanding the past and present; it's about anticipating the future and prescribing optimal courses of action. This advanced approach is becoming increasingly essential as businesses navigate an environment characterized by vast data volumes, rapid change, and a need for proactive decision-making.
At the heart of AI-driven analytics is machine learning, which enables systems to learn from data without being explicitly programmed. Machine learning algorithms can identify patterns, make predictions, and improve their performance over time as they are exposed to more data. Deep learning, a subset of machine learning, utilizes artificial neural networks with multiple layers to analyze complex data representations, enabling breakthroughs in areas like image and speech recognition, and advanced predictive modeling. Natural Language Processing (NLP) empowers systems to 'understand', interpret, and generate human language, allowing for the analysis of unstructured text data from sources like customer feedback, social media, and documents. Predictive analytics uses these AI techniques to forecast future outcomes based on historical data patterns, while prescriptive analytics goes a step further by recommending actions to optimize business objectives. Platforms like Google Vertex AI, AWS SageMaker, and Azure Machine Learning provide comprehensive environments for developing and deploying AI-driven analytics solutions.
The applications of AI-driven analytics are transformative across industries. In marketing, AI powers personalized customer experiences, targeted advertising, and churn prediction. In finance, it's used for fraud detection, algorithmic trading, and risk management. Healthcare benefits from AI in diagnostic imaging, personalized medicine, and drug discovery. Manufacturing leverages AI for predictive maintenance, quality control, and supply chain optimization. Retail utilizes AI for demand forecasting, inventory management, and customer sentiment analysis. The ability of AI to process vast amounts of data, uncover hidden relationships, and provide forward-looking insights opens up new frontiers for innovation and competitive advantage in virtually every sector.
Strengths of AI-Driven Analytics
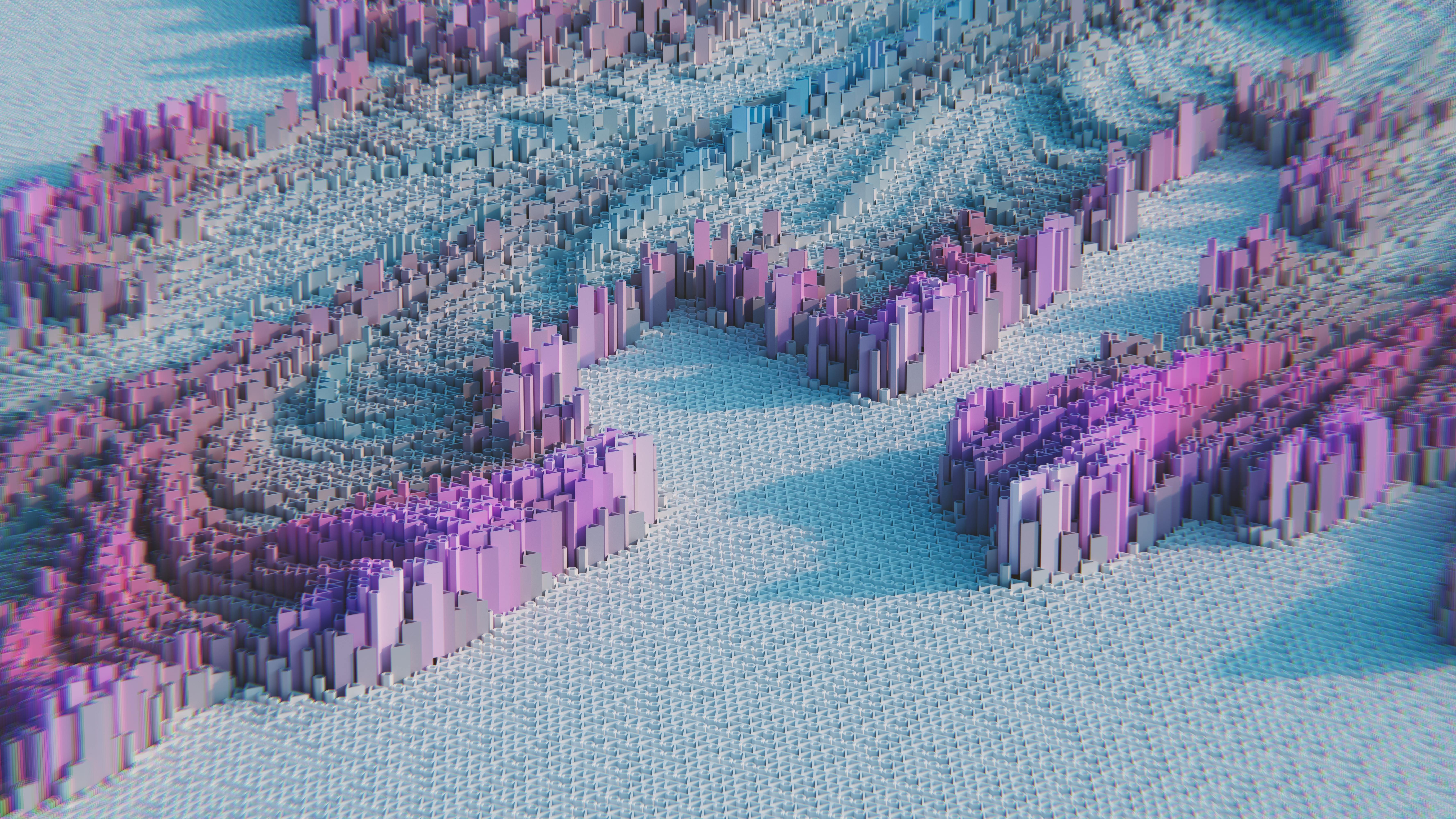
- Ability to Handle Big Data and Unstructured Data: AI-driven analytics excels in processing and analyzing the massive volumes of data characteristic of the big data era. Machine learning and deep learning algorithms are designed to handle large datasets efficiently, extracting meaningful insights even from complex and high-dimensional data. Furthermore, AI is particularly adept at analyzing unstructured data, such as text, images, audio, and video, which traditional analytics often struggles with. For example, NLP techniques can extract sentiment and topics from customer reviews, while computer vision can analyze images for quality control in manufacturing. This capability to handle diverse data types and large volumes allows businesses to unlock insights from previously untapped data sources.
- Predictive Capabilities and Real-Time Analysis: A significant advantage of AI-driven analytics is its predictive power. Machine learning models can be trained to forecast future trends, predict customer behavior, and anticipate potential risks with a high degree of accuracy. This predictive capability enables proactive decision-making, allowing businesses to anticipate market changes, optimize resource allocation, and mitigate potential problems before they occur. Moreover, AI can process data in real-time or near real-time, providing instant insights for immediate action. This real-time analytical capability is crucial for applications like fraud detection, dynamic pricing, and personalized recommendations, where timely responses are essential.
- Potential for Uncovering Hidden Patterns and Insights: AI algorithms can identify complex patterns and relationships in data that might be too subtle or intricate for traditional analytical methods to detect. Machine learning models can uncover non-linear relationships, interactions between variables, and anomalies that are not readily apparent through standard statistical techniques. This ability to discover hidden insights can lead to breakthrough innovations, new business opportunities, and a deeper understanding of complex phenomena. For example, AI can analyze customer behavior data to identify previously unknown customer segments or predict emerging market trends that traditional market research might miss.
Weaknesses of AI-Driven Analytics
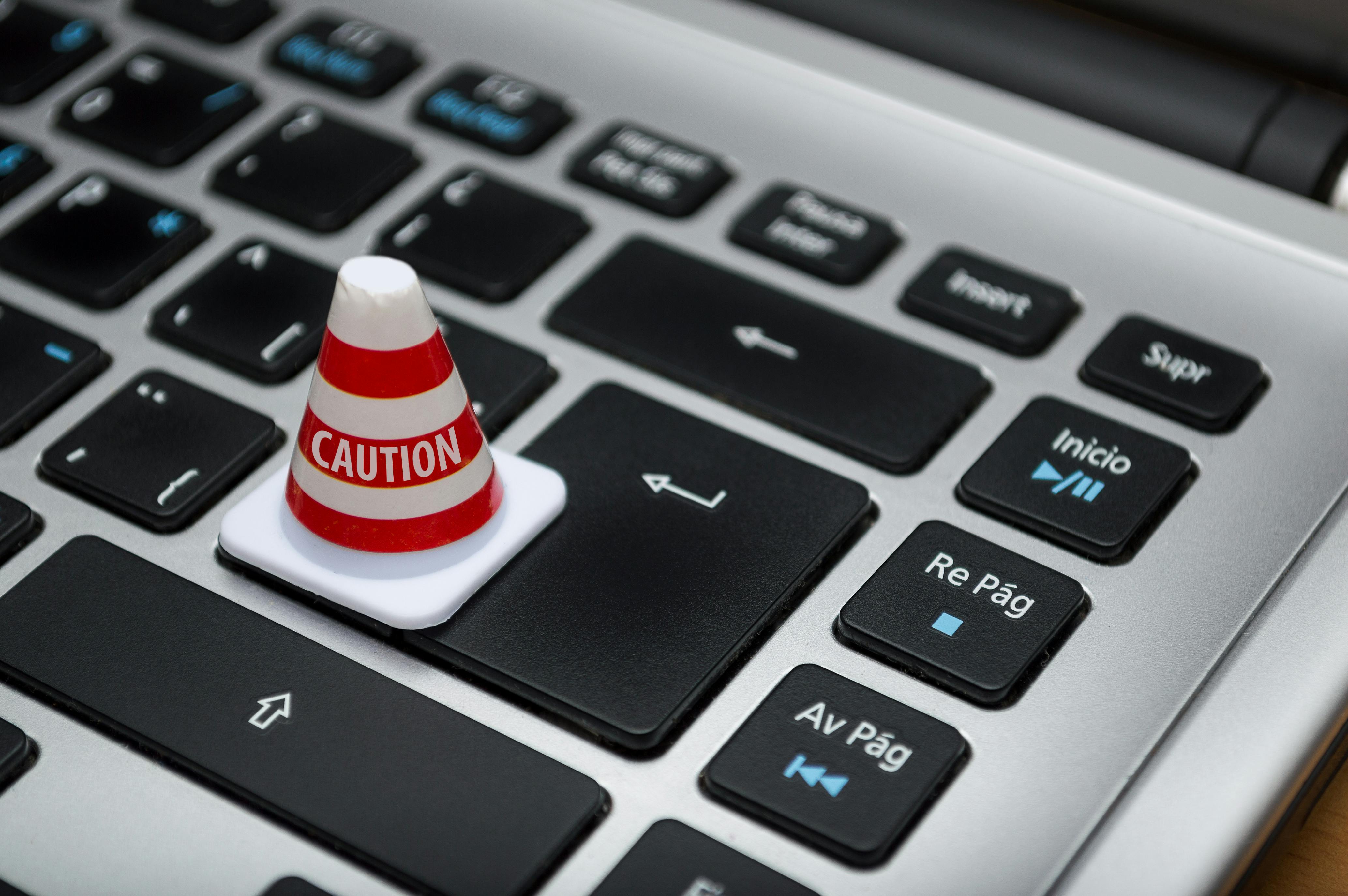
- Complexity and Cost of Implementation: Implementing AI-driven analytics solutions can be significantly more complex and costly compared to traditional analytics. AI projects often require specialized infrastructure, including powerful computing resources and cloud-based platforms. Developing and deploying AI models demands expertise in data science, machine learning, and software engineering, which can be expensive to acquire or develop in-house. Furthermore, the iterative nature of AI model development, including data preparation, model training, and validation, can be time-consuming and resource-intensive. While the long-term benefits of AI can outweigh the initial costs, the upfront investment and ongoing operational expenses can be a barrier for some organizations, especially smaller businesses with limited resources.
- Issues with Data Privacy and Ethical Concerns: AI-driven analytics raises significant data privacy and ethical concerns. Many AI models, particularly deep learning models, require vast amounts of data for training, which may include sensitive personal information. The use of AI in decision-making can also lead to ethical dilemmas, such as algorithmic bias, fairness issues, and lack of transparency. Ensuring data privacy, complying with regulations like GDPR and CCPA, and addressing ethical considerations in AI development and deployment are critical challenges. Organizations must implement robust data governance policies, anonymization techniques, and ethical frameworks to mitigate these risks and build trust in AI-driven systems. Learn more about data governance here.
- Need for Specialized Skills and Knowledge: Successful implementation of AI-driven analytics requires specialized skills and knowledge that may not be readily available within traditional business teams. Data scientists, machine learning engineers, NLP specialists, and AI ethicists are increasingly in demand, and finding and retaining talent in these fields can be challenging and expensive. Training existing staff to acquire these skills requires significant investment in time and resources. The skills gap in AI is a major obstacle for many organizations seeking to adopt AI-driven analytics, highlighting the need for strategic workforce planning and investment in specialized training programs.
Complementary Roles of AI and Traditional Analytics
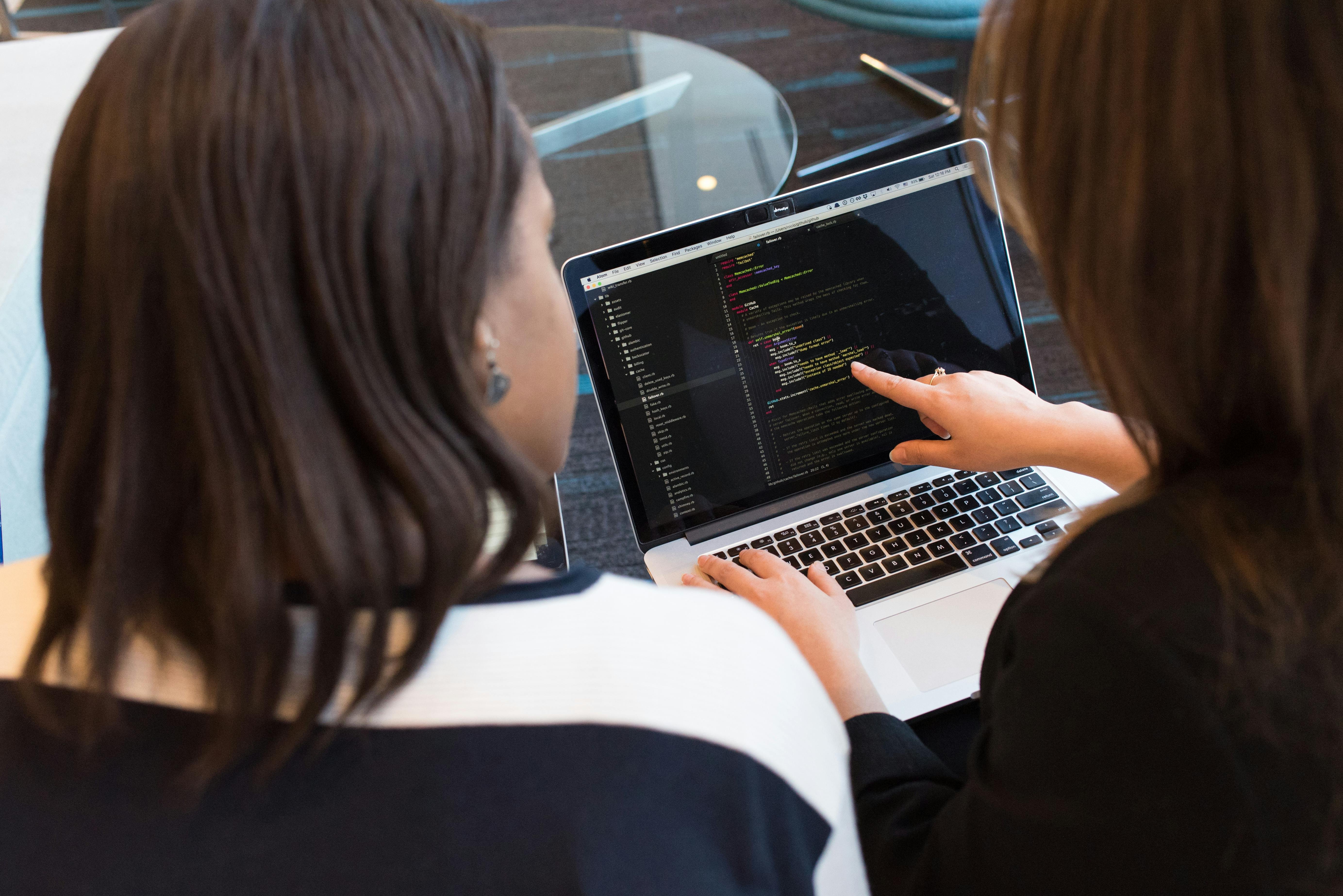
While AI-driven analytics offers advanced capabilities, it is not intended to replace traditional analytics entirely. In fact, the most effective data strategies often involve a synergistic approach where AI and traditional analytics work together, each complementing the strengths and mitigating the weaknesses of the other. This blended approach allows organizations to gain a more comprehensive and nuanced understanding of their data, leading to more robust insights and better decision-making. The key lies in recognizing where each approach is most effective and strategically integrating them to maximize analytical power.
How Traditional Analytics and AI Can Work Together
- Traditional Analytics Provides a Foundation for AI: Traditional analytics often serves as a crucial foundation for successful AI initiatives. Before deploying complex AI models, it is essential to have a solid understanding of the data landscape, data quality, and basic trends. Traditional descriptive analytics helps in data exploration, cleaning, and preprocessing, which are critical steps in preparing data for AI model training. For example, understanding data distributions, identifying outliers, and ensuring data consistency through traditional methods lays the groundwork for building more accurate and reliable AI models. Furthermore, traditional analytics can help define the business problem and formulate hypotheses that AI can then explore and validate at a deeper level.
- AI Enhances Insights from Traditional Methods: AI can significantly enhance the insights gained from traditional analytics by adding layers of sophistication and depth. While traditional analytics can identify trends and correlations, AI can uncover more complex patterns, non-linear relationships, and hidden anomalies that traditional methods might miss. For instance, traditional regression analysis can identify the general relationship between marketing spend and sales, but AI-powered machine learning can model this relationship with greater accuracy by considering a wider range of variables and their interactions, leading to more precise marketing ROI predictions. Similarly, AI can automate and scale traditional analytical tasks, such as report generation and data visualization, freeing up analysts to focus on higher-level interpretation and strategic thinking.
- Examples of Successful Integration: Many businesses are successfully integrating AI and traditional analytics to achieve superior analytical outcomes. In customer relationship management (CRM), traditional analytics can segment customers based on demographics and purchase history, while AI can enhance this segmentation by predicting customer churn risk and personalizing marketing messages based on real-time behavior. In supply chain management, traditional analytics can monitor inventory levels and track delivery times, while AI can predict demand fluctuations, optimize logistics routes, and proactively identify potential supply chain disruptions. In fraud detection, traditional rule-based systems can flag suspicious transactions based on predefined criteria, while AI can detect more sophisticated fraud patterns by learning from vast transaction datasets and adapting to evolving fraud techniques. These examples demonstrate how the combination of traditional and AI-driven analytics can lead to more robust, comprehensive, and actionable insights across various business functions.
Case Studies
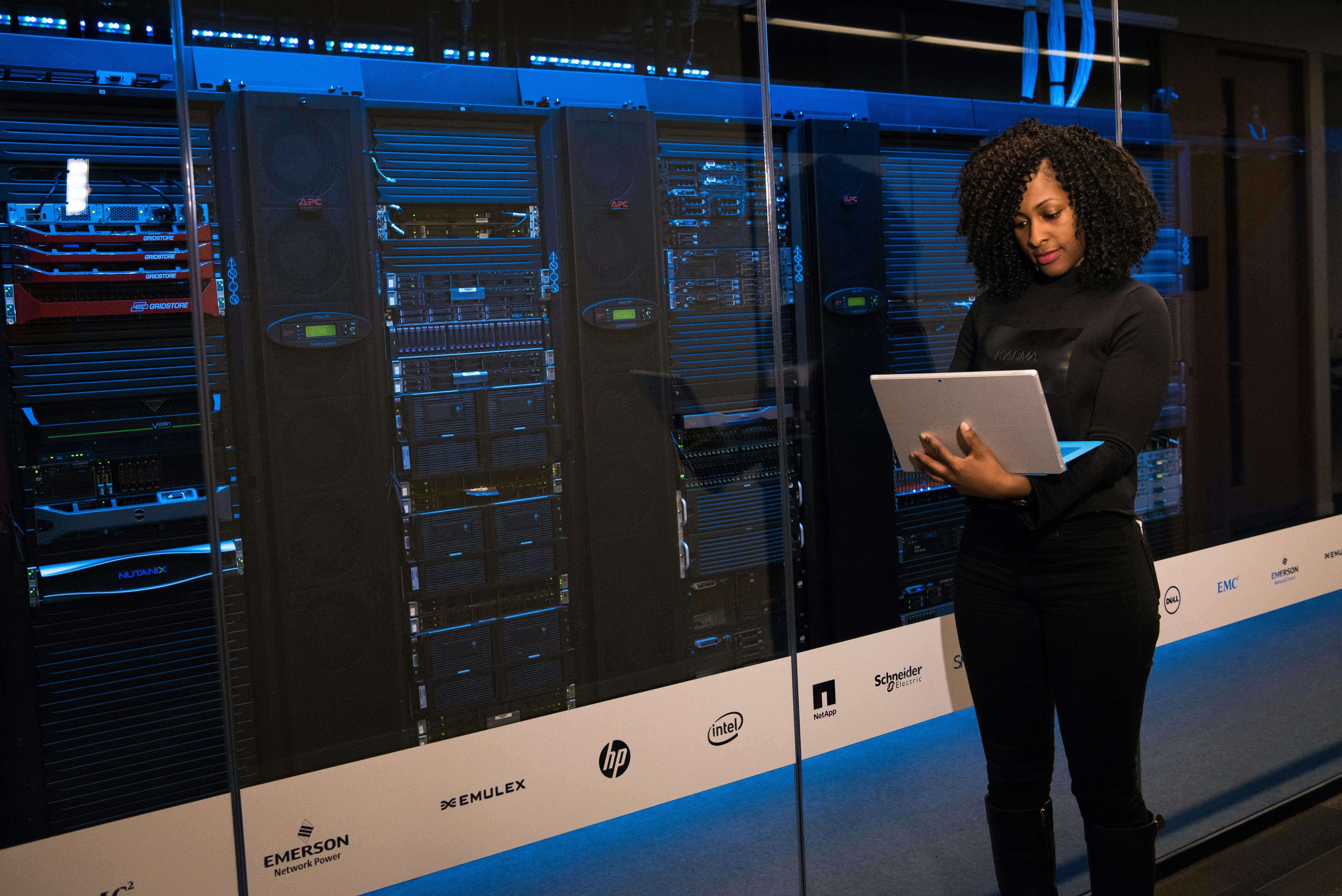
To illustrate the practical benefits of combining AI and traditional analytics, let's examine a few hypothetical case studies across different industries:
- Case Study 1: Retail - Personalized Customer Experience: A large online retailer utilizes traditional analytics to segment customers based on basic demographics and purchase history, creating broad customer groups for targeted marketing campaigns. Simultaneously, they implement an AI-driven recommendation engine that analyzes real-time browsing behavior, past purchases, and product reviews to provide highly personalized product recommendations to each individual customer. Traditional analytics provides the foundational customer segmentation, while AI enhances the customer experience by offering dynamic and personalized product suggestions, leading to increased sales and customer satisfaction. The retailer also uses traditional A/B testing methodologies to validate the uplift from the AI recommendations.
- Case Study 2: Manufacturing - Predictive Maintenance: A manufacturing company employs traditional statistical process control (SPC) charts to monitor equipment performance and detect deviations from normal operating ranges. They also integrate AI-powered predictive maintenance models that analyze sensor data from machinery, historical maintenance records, and environmental factors to predict potential equipment failures before they occur. Traditional SPC provides immediate alerts for current anomalies, while AI proactively forecasts future maintenance needs, allowing for scheduled maintenance, reduced downtime, and optimized equipment lifespan. The company uses traditional cost-benefit analysis to demonstrate the ROI of the AI-driven predictive maintenance system compared to reactive maintenance strategies.
- Case Study 3: Healthcare - Patient Care Optimization: A hospital uses traditional electronic health record (EHR) analysis to track patient demographics, medical history, and treatment outcomes, generating reports for population health management. They also implement AI-driven diagnostic tools that analyze medical images (X-rays, MRIs) and patient symptoms to assist physicians in making more accurate and timely diagnoses. Traditional EHR analytics provides a broad overview of patient populations and treatment trends, while AI enhances diagnostic accuracy and speed, potentially improving patient outcomes and reducing diagnostic errors. The hospital uses traditional clinical trials and statistical validation methods to ensure the safety and efficacy of AI-assisted diagnostic tools.
These case studies highlight that the combined approach of traditional and AI-driven analytics provides a more robust and versatile analytical capability. Traditional methods provide structure, interpretability, and validation, while AI adds depth, predictive power, and the ability to handle complex data.
Practical Steps for Integrating AI and Traditional Analytics
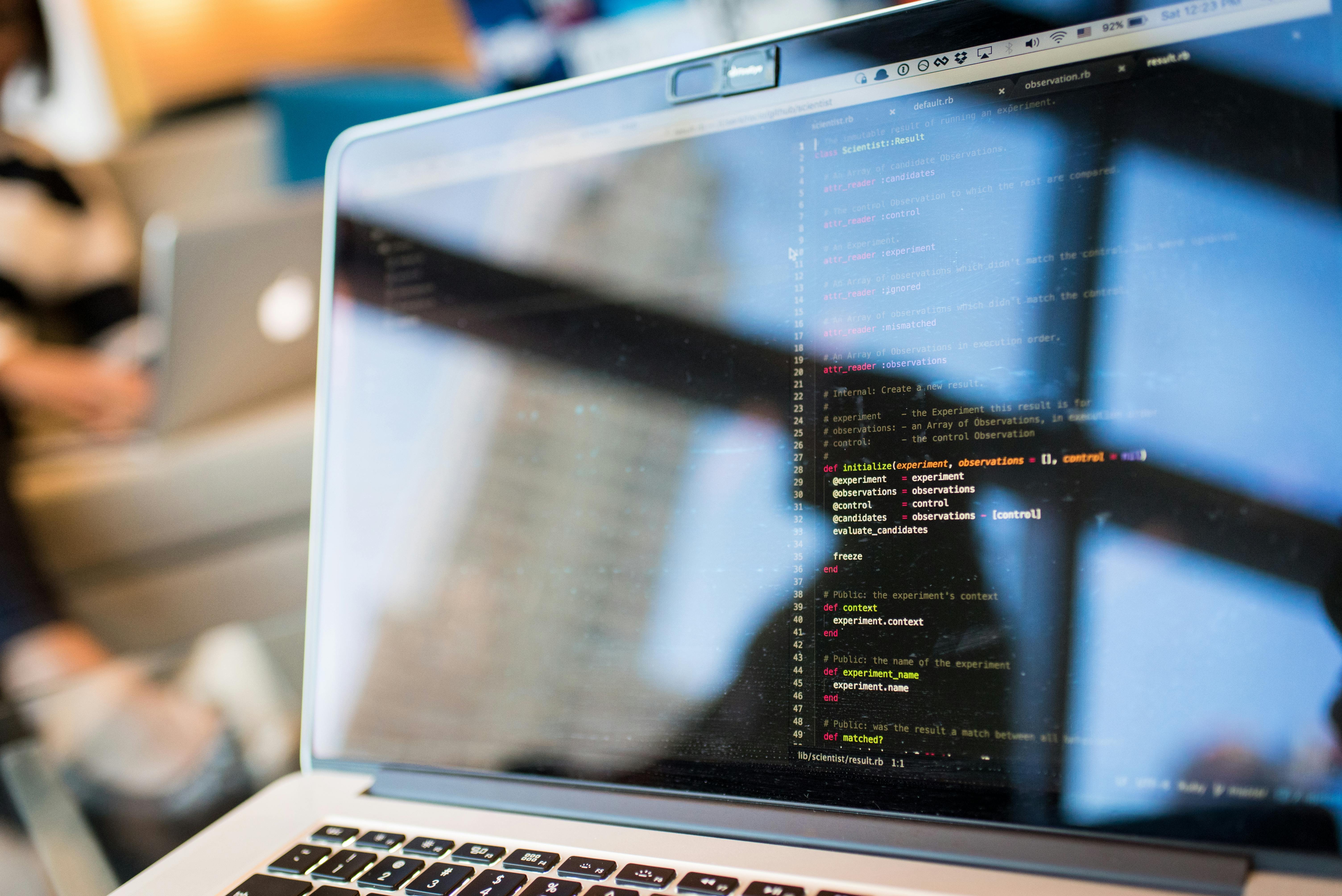
Integrating AI and traditional analytics is not an overnight process; it's a strategic journey that requires careful planning, phased implementation, and a commitment to continuous improvement. Organizations should approach this integration systematically, starting with a clear understanding of their business needs and existing analytical capabilities, and gradually incorporating AI in a way that complements and enhances their traditional analytics framework. A step-by-step approach ensures a smoother transition, minimizes disruption, and maximizes the return on investment in both traditional and AI-driven analytics.
Assessing Your Business Needs
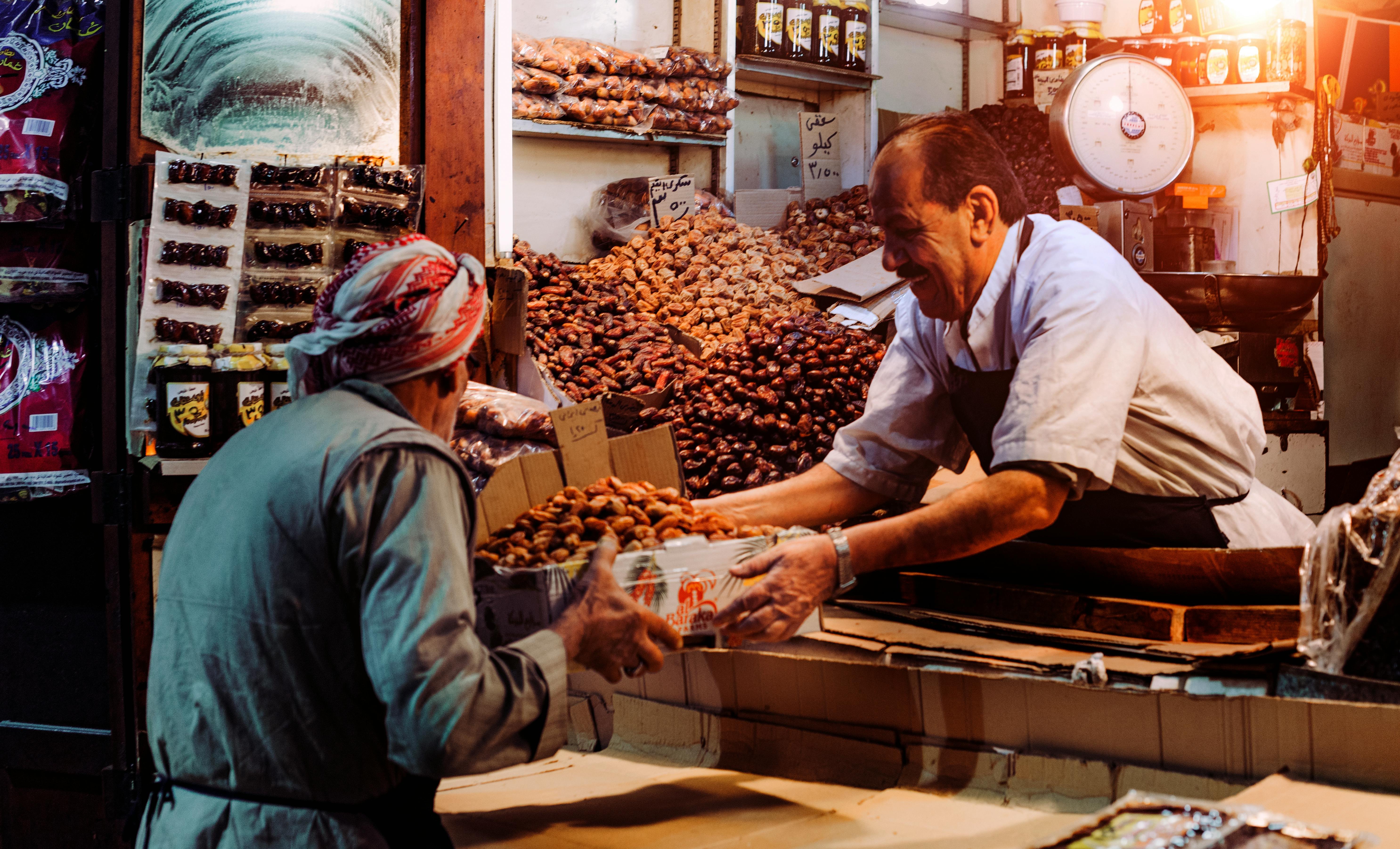
- Evaluate Current Analytics Capabilities: The first step is to conduct a thorough assessment of your organization’s current analytics capabilities. This involves understanding what data you collect, how it is stored and processed, what traditional analytics tools and techniques are currently in use, and what skills and expertise your team possesses. Identify the strengths and weaknesses of your existing analytics infrastructure. Are you effectively leveraging descriptive statistics and data visualization? Are your current systems capable of handling real-time data or large datasets? This assessment will provide a baseline for identifying gaps and opportunities for improvement through the integration of AI.
- Identify Key Business Questions: Clearly define the key business questions that need answering through data analytics. What are your strategic objectives? What operational challenges are you facing? What insights are critical for driving growth, efficiency, or innovation? Frame these questions in a way that is specific, measurable, achievable, relevant, and time-bound (SMART). For example, instead of asking "How can we improve sales?", ask "How can we increase online sales by 15% in the next quarter?". Prioritize these business questions based on their potential impact and alignment with your strategic goals. This focused approach will guide the selection of appropriate analytical techniques, whether traditional or AI-driven, and ensure that your analytics efforts are directly contributing to business value.
Building a Balanced Analytics Strategy
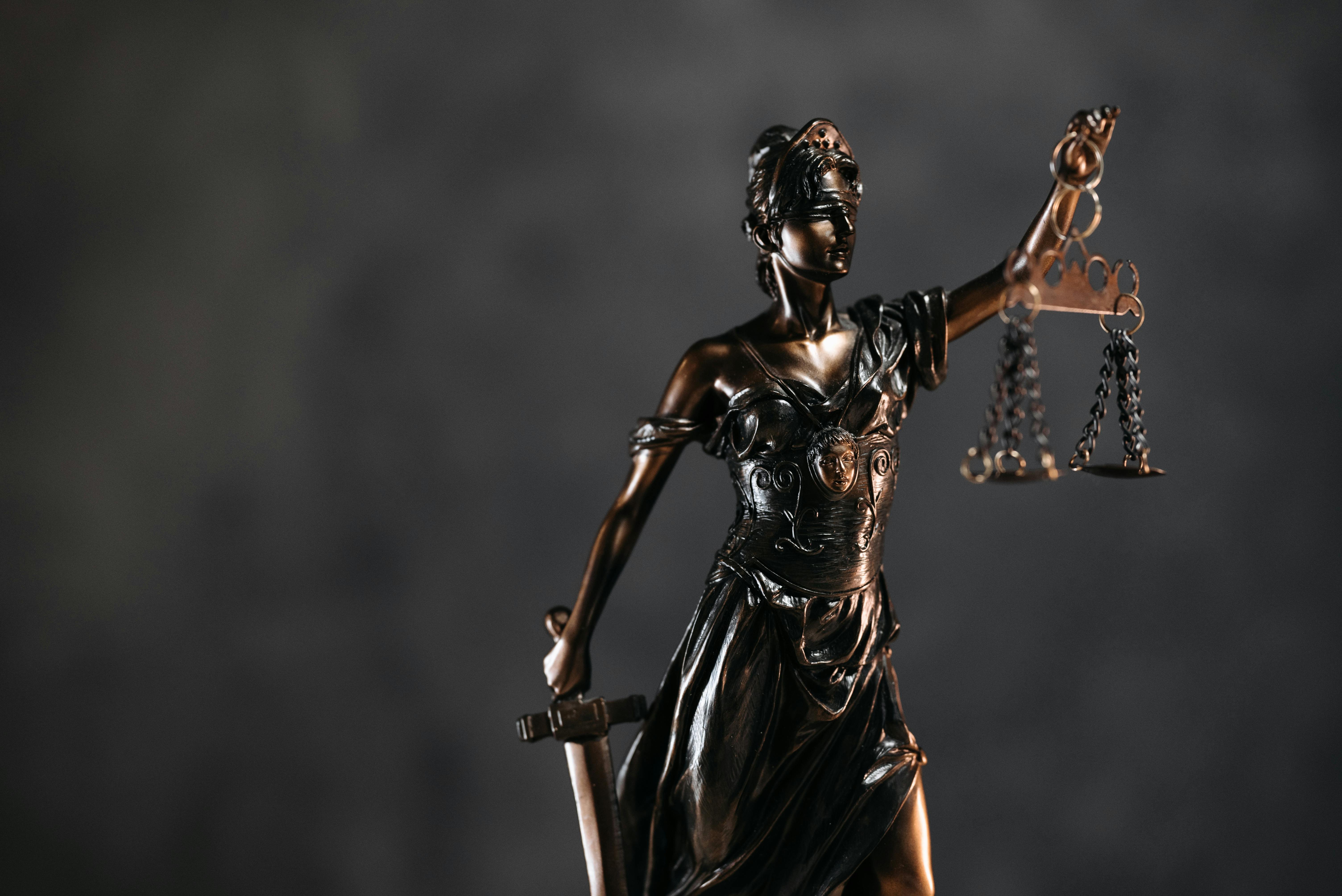
- Start with Traditional Analytics and Gradually Incorporate AI: For many organizations, especially those new to advanced analytics, a phased approach is recommended. Begin by strengthening your foundation in traditional analytics. Implement robust data collection and storage processes, establish data governance policies, and train your team in basic statistical analysis and data visualization techniques. Once you have a solid understanding of your data and are effectively using traditional methods to address business questions, start exploring opportunities to incorporate AI. Identify specific areas where AI can provide incremental value, such as predictive modeling, anomaly detection, or natural language processing. Gradually introduce AI tools and techniques, starting with pilot projects and scaling up as you gain experience and demonstrate success. This incremental approach minimizes risk, allows for learning and adaptation, and ensures a smoother integration process.
- Invest in Training Staff and the Right Tools: Successful integration of AI and traditional analytics requires investment in both human capital and technology. Provide training to your staff to enhance their skills in both traditional and AI-driven analytics. This may include training in statistical software, data visualization tools, machine learning platforms, and AI ethics. Consider both internal training programs and external professional development opportunities. Simultaneously, invest in the right analytical tools and platforms. This may involve upgrading your existing traditional BI tools, adopting cloud-based data warehousing solutions, and selecting AI platforms that align with your business needs and technical capabilities. Choose tools that are user-friendly, scalable, and interoperable, and ensure that your team is adequately trained to use them effectively.
Overcoming Challenges
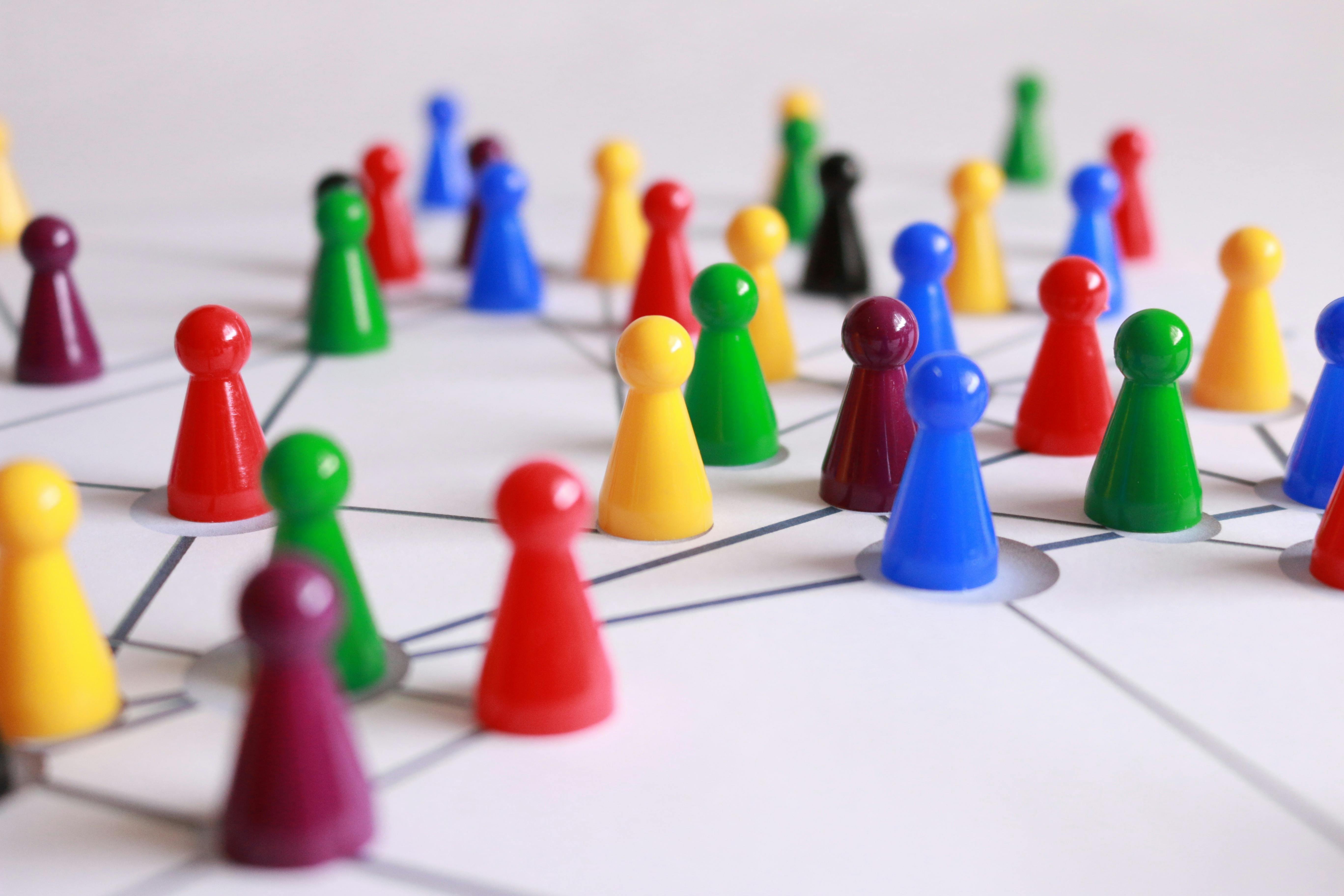
- Address Data Silos and Data Quality Issues: Data silos and poor data quality are significant challenges that can hinder both traditional and AI-driven analytics. Before integrating AI, address data silos by implementing data integration strategies, such as establishing a centralized data repository or utilizing data virtualization techniques. Improve data quality by implementing data governance policies, data cleansing processes, and data validation procedures. Ensure that data is accurate, consistent, complete, and timely. High-quality, integrated data is essential for both reliable traditional analytics and effective AI model training and performance. Learn more about breaking down data silos here.
- Establish Data Governance and Ethical Considerations: As you integrate AI, establish robust data governance frameworks and address ethical considerations proactively. Define clear roles and responsibilities for data management, access, and security. Implement data privacy policies and comply with relevant regulations. Develop ethical guidelines for AI development and deployment, addressing issues such as algorithmic bias, fairness, transparency, and accountability. Establish mechanisms for monitoring and auditing AI systems to ensure ethical and responsible use. Data governance and ethical frameworks are crucial for building trust in both traditional and AI-driven analytics and ensuring long-term sustainability.
Conclusion
In conclusion, both AI-driven and traditional analytics offer unique strengths and address different aspects of data analysis. Traditional analytics provides a robust foundation for understanding historical data, identifying trends, and making informed decisions based on established methodologies. Its strengths lie in its reliability, interpretability, and cost-effectiveness, making it accessible and valuable for businesses of all sizes. However, traditional analytics has limitations in handling large datasets, adapting to real-time analysis, and uncovering complex patterns.
AI-driven analytics, on the other hand, excels in processing big data, analyzing unstructured data, and providing predictive and prescriptive insights. Its strengths include the ability to uncover hidden patterns, forecast future outcomes, and automate complex analytical tasks. However, AI comes with its own set of challenges, including complexity, cost, ethical concerns, and the need for specialized skills.
The optimal approach for most organizations is not to choose between AI and traditional analytics, but to strategically integrate both. Traditional analytics provides the essential groundwork, data understanding, and validation, while AI enhances analytical capabilities with predictive power, deeper insights, and real-time processing. By combining these approaches, businesses can create a more balanced and powerful analytics strategy, leveraging the strengths of each to overcome their respective weaknesses. The synergistic combination of AI and traditional analytics empowers organizations to gain a more comprehensive, nuanced, and actionable understanding of their data, leading to better decision-making, improved operational efficiency, and a stronger competitive advantage in the data-driven era.
Ultimately, the key to successful data analytics lies in understanding your business needs, assessing your current capabilities, and building a balanced strategy that leverages both traditional and AI-driven approaches. By embracing this complementary relationship, organizations can unlock the full potential of their data and embark on a journey of continuous improvement and data-driven innovation.
What next?
Ready to take the next step in integrating AI and traditional analytics within your organization? Explore the resources available on our website to equip your teams with the knowledge and tools they need. Share this guide with your colleagues or on social media to spark conversations and encourage a balanced approach to data analytics in your workplace.
Remember: building a robust and integrated analytics capability is a journey, not a destination. Start by assessing your current state, defining your business needs, and gradually incorporating AI in a way that complements your existing traditional analytics framework. Focus on building a strong data foundation, investing in training and tools, and addressing ethical considerations proactively. Each step you take will bring you closer to unlocking the full potential of your data and achieving data-driven excellence.
References and Further Reading
Below is a curated list of resources for those eager to deepen their understanding of AI-driven and traditional analytics, and best practices for building a data-driven organization:
- Provost, F., & Fawcett, T. (2013). Data Science for Business: What You Need to Know About Data Mining and Data-Analytic Thinking. O'Reilly Media. - A comprehensive guide to data science principles and their application in business.
- Shmueli, G., Patel, N. R., & Bruce, P. C. (2017). Data Mining for Business Analytics: Concepts, Techniques, and Applications in Python. Wiley. - A practical guide to data mining techniques with a focus on business applications.
- Goodfellow, I., Bengio, Y., & Courville, A. (2016). Deep Learning. MIT Press. - A foundational textbook on deep learning, covering the theoretical underpinnings and practical applications.
- Russell, S. J., & Norvig, P. (2020). Artificial Intelligence: A Modern Approach. Pearson. - A comprehensive textbook on artificial intelligence, covering a wide range of AI topics, including machine learning and natural language processing.
- Manyika, J., Chui, M., Brown, B., Bughin, J., Dobrin, R., Roxburgh, C., & Byers, A. H. (2011). Big data: The next frontier for innovation, competition, and productivity. McKinsey Global Institute. - A report by McKinsey Global Institute on the impact of big data on innovation and productivity.